YANG Xing. Compressive imaging based on Tetrolet-domain uHMT structured sparse prior and Turbo equalization[J]. Optics and Precision Engineering, 2018, 26(7): 1766

Search by keywords or author
- Optics and Precision Engineering
- Vol. 26, Issue 7, 1766 (2018)
Abstract
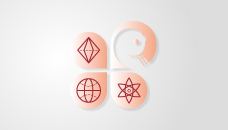
Set citation alerts for the article
Please enter your email address