Carlo M. Valensise, Ivana Grecco, Davide Pierangeli, Claudio Conti, "Large-scale photonic natural language processing," Photonics Res. 10, 2846 (2022)

Search by keywords or author
- Photonics Research
- Vol. 10, Issue 12, 2846 (2022)
![Three-dimensional PELM for language processing. (A) The text database entry is a paragraph of variable length. Text pre-processing: a sparse representation of the input paragraph is mapped into a Hadamard matrix with phase values in [0,π]. (B) The mask is encoded into the optical wavefront by a phase-only SLM. Free-space propagation of the optical field maps the input data into a 3D intensity distribution (speckle-like volume). (C) Sampling the propagating laser beam in multiple far-field planes enables upscaling the feature space. Intensities picked from all the spatial modes form the output layer H3D that undergoes training via ridge regression. By using three planes (j=3), we get a network capacity C>1010. (D) The example shows a binary text classification problem for large-scale rating.](/richHtml/prj/2022/10/12/2846/img_001.jpg)
Fig. 1. Three-dimensional PELM for language processing. (A) The text database entry is a paragraph of variable length. Text pre-processing: a sparse representation of the input paragraph is mapped into a Hadamard matrix with phase values in [ 0 , π ] . (B) The mask is encoded into the optical wavefront by a phase-only SLM. Free-space propagation of the optical field maps the input data into a 3D intensity distribution (speckle-like volume). (C) Sampling the propagating laser beam in multiple far-field planes enables upscaling the feature space. Intensities picked from all the spatial modes form the output layer H 3 D that undergoes training via ridge regression. By using three planes (j = 3 ), we get a network capacity C > 10 10 . (D) The example shows a binary text classification problem for large-scale rating.
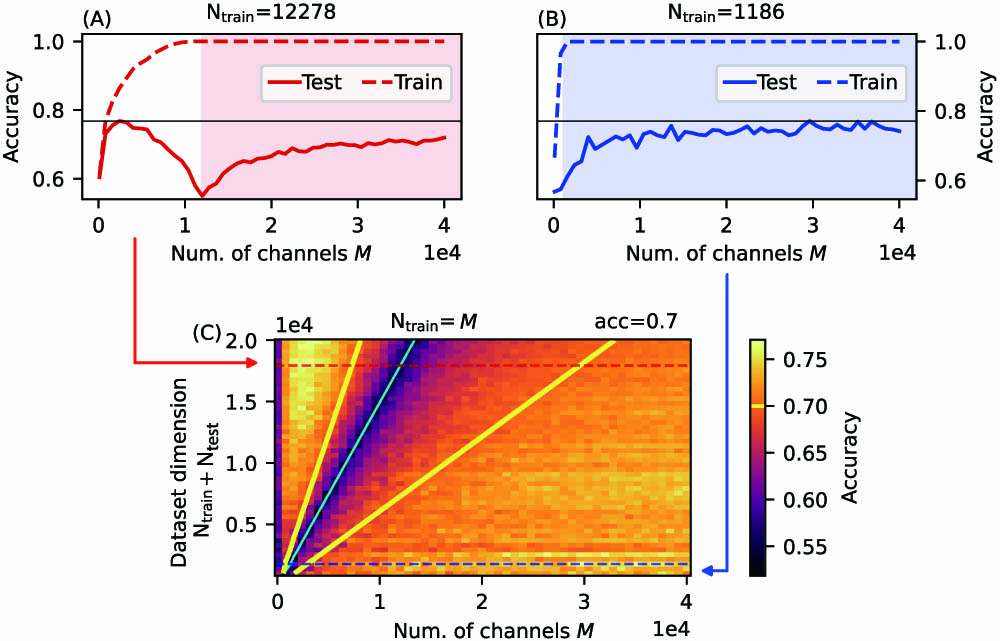
Fig. 2. Photonic sentiment analysis. (A), (B) Training and test accuracy of the 3D-PELM on the IMDb dataset as a function of the number of output channels. The shaded area corresponds to the over-parameterized region. The configuration in (B) allows us to reach very high accuracy in the over-parameterized region with a dataset limited to N train = 1186 training points. In (A), the same accuracy is reached in the under-parameterized region with N train = 12 , 278 . Black horizontal lines correspond to the maximum test accuracy achieved (0.77). (C) IMDb classification accuracy by varying the number of features M and training dataset size N train . The boundary between the under and over-parameterized region (interpolation threshold), N train = M , is characterized by a sharp accuracy drop (cyan contour line).
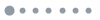
Fig. 3. Performances at ultralarge scale. (A)–(C) Test accuracy as a function of M for different input sizes L . In all cases, the 3D-PELM performance saturates in the over-parameterized region, reaching a plateau. A linear fit of the data preceding the plateau shows that the onset of the saturation is faster for datasets with a larger input space. The corresponding angular coefficient m is inset in each panel. (D) Test accuracy varying the training set size for M = 0.8 × 10 5 and M = 1.2 × 10 5 .
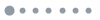
Fig. 4. Analysis of the IMDb accuracy. (A), (B) The comparison reports the accuracy for the experimental device (3D-PELM device), the simulated device (3D-PELM numerics), the random projection method with ridge regression (RP), the support vector machine (SVM), and a convolutional neural network (CNN) in both the under-parameterized (M = 1 × 10 3 ) and over-parameterized (M = 4 × 10 4 ) regimes, for (A) N train = 6700 and (B) N train = 1500 . 8-bit numerical results, when applicable, refer to the over-parameterized regime.
|
Table 1. Maximum Network Capacity of Current Photonic Neuromorphic Computing Hardware for Supervised Learning
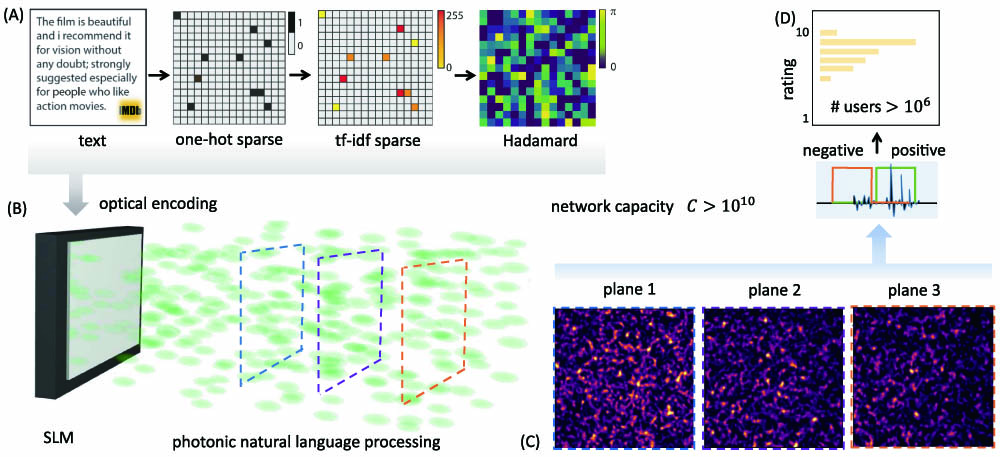
Set citation alerts for the article
Please enter your email address