ZHAO Peng, LU Zhi, JIANG Zhen-hua, QU Xiao-ping, WU Yi-nong. Cooling Performance Prediction Model of Pulse Tube Cryocooler Based on Random Forest Regression Analysis[J]. INFRARED, 2021, 42(8): 33

Search by keywords or author
- INFRARED
- Vol. 42, Issue 8, 33 (2021)
Abstract
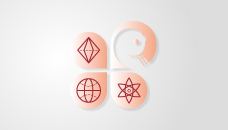
Set citation alerts for the article
Please enter your email address