ZHANG Wenbo, QU Jue, WANG Wei, HU Jun, WANG Qingli. An Improved Deeplab v3+ Image Semantic Segmentation Algorithm Incorporating Multi-Scale Features[J]. Electronics Optics & Control, 2022, 29(11): 12

Search by keywords or author
- Electronics Optics & Control
- Vol. 29, Issue 11, 12 (2022)
Abstract
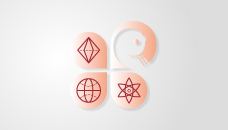
Set citation alerts for the article
Please enter your email address