CHENG Baozhi, ZHANG Lili, ZHAO Chunhui. Joint Low-Rank Tensor Decomposition and Sparse Representation of Anomaly Target Detection for Hyperspectral Imagery[J]. Electronics Optics & Control, 2023, 30(1): 57

Search by keywords or author
- Electronics Optics & Control
- Vol. 30, Issue 1, 57 (2023)
Abstract
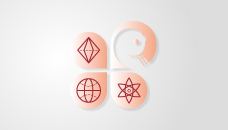
Set citation alerts for the article
Please enter your email address