
- Chinese Optics Letters
- Vol. 13, Issue 2, 021405 (2015)
Abstract
Unbalanced random numbers (URNs) are required in some selection processes. URNs, compared with balanced random numbers (BRNs), are those numbers that bit “0” and “1” as occupying different proportions in the overall data while keep the random property of unpredictability at the same time. One recent application of URNs is in quantum key distribution (QKD)[
At present, researchers mainly use pseudo-URNs in applications that require URNs. The pseudo-URNs are produced by deterministic unbalanced random-number generators (URNGs) based on the computational complexity of the algorithms. This means that once given the initial conditions of the algorithm, the sequence produced by such URNGs is determined. Thus it cannot meet the randomness requirement of complete unpredictability. On the other hand, nondeterministic URNGs based on stochastic physical processes can produce true URNs which can satisfy the security requirement mentioned previously. This kind of URNG can be further divided into two types according to the scheme of generating URNs. The first type is based on nondeterministic balanced random-number generators (BRNGs). This type of nondeterministic URNG produce the required URNs through transforming the BRNs generated from BRNGs with appropriate algorithms. The second type of nondeterministic URNG is those random-number generators (RNGs) that based on stochastic physical processes and can produce URNs directly without transfoming.
The first type of nondeterministic URNGs is proposed since the technology of nondeterministic BRNGs gradually tends to be mature nowadays. In past decades, researchers have brought up balanced physical RNGs based on stochastic physical random processes such as radioactive decay[
Sign up for Chinese Optics Letters TOC. Get the latest issue of Chinese Optics Letters delivered right to you!Sign up now
To illustrate the low efficiency and discontinuous performance of the this type of URNG, we introduce a conventional transforming algorithm, a deformation of the Von Neumann (VN) unbias algorithm that can transform the BRNs into required URNs. The original VN unbias algorithm can be used to transform an biased sequence of BRNs into a shorter unbiased BRNs. After distorting, this algorithm is suitable to meet the application of transforming a sequence of BRNs into a shorter sequence of URNs with the required ratio between “1” and “0.” To achieve this, we describe the deformation process as follows.
We analyze the efficiency of the algorithm in Fig.
Figure 1.Efficiency of extracting bits from raw unbiased random sequence.
In this Letter, we proposed an adjustable unbalanced quantum random number generator (UQRNG) of the second type. Quantum random number generators (QRNGs) are those nondeterministic RNGs that rely on fundamental quantum principles. The proposed UQRNG is based on the quantum superposition principle that the quantum state of photon collapses to a certain direction at a certain probability. The advantage of the proposed QRNG is that it can produce URNs much intuitively with high efficiency and can continuously cover all required probabilities. The experimental demonstration of the proposed UQRNG is shown in Fig.
Figure 2.Experimental setup.
The proposed system also employs a circuit feedback system with a polarization control algorithm in the experimental setup. The polarization control algorithm is a gradient algorithm. The input of the algorithm is the ratio between the statistical detect events of SPD1 and SPD2 in 1 s. According to the ratio, the control algorithm will adjust the EPC to achieve the ratio desired. The EPC performs a live verification of the ratio between counts of SPD1 and SPD2 every 5 min. This ratio is the result of the joint action of PBS and different detection efficiencies of two detectors. The EPC checks whether this joint action is rigorously working at the required value through a feedback of the ratio of the output stream statistics of SPD1 and SPD2. If the proportion of “1” is within 1% error from the desired proportion, the bit stream is accepted. Otherwise, the EPC adjusts the photon polarization state to make the ratio achieve the required value and the bit stream obtained within this period of time is abandoned.
In this work, we apply a light source with repetition frequency of 1 MHz. The light source is attenuated to 0.1 photon/pulse and the detection efficiency of the two SPDs is 10%. Thus, the URNs can be generated at a rate of magnitude
The next step is estimating the randomness of the output URNs produced by this system. A sequence of BRNs is random if it obeys perfect uniform distribution, as it means completely unpredictable. Similarly to this, the randomness of URN sequence is judged by whether it obeys perfect unbalanced “uniform” distribution. Furthermore, the distribution of a sequence can be displayed by the min-entropy of the sequence[
The bias means the ratio between bit “1” and “0” of the obtained random sequence is not exactly what we desired. In this work, since the EPC is adjusted to set the mark ratio to the required ratio through a feedback of the ratio of the output stream statistics of SPD1 and SPD2, the accuracy of the required probability of “0” and “1” can reach high precision. On the other hand, the correlations between adjacent bits are caused by the after pulses of the SPDs. This means if a click occurs in one detector, it is more likely to be followed by a click in this detector again than the other, which constitutes a correlation.
Due to the difference of test standards, the randomness of the output RNs is discussed dividing it into two categories (BRNs and URNs).
For the BRNs (
The autocorrelation coefficient
Figure 3.(a) Bias
The NIST test suite can better qualify the statistical randomness of the generated BRNs of our generator. The typical test result of 1 Gb BRNs is shown in Table
Statistical Test | Proportion | Result | |
---|---|---|---|
Frequency | 0.476911 | 0.9920 | Success |
Block frequency | 0.123755 | 0.9890 | Success |
Cumulative sums | 0.382115 | 0.9900 | Success |
Runs | 0.348869 | 0.9860 | Success |
Longest run | 0.670396 | 0.9940 | Success |
Rank | 0.816537 | 0.9820 | Success |
Spectral | 0.078086 | 0.9870 | Success |
Nonoverlapping template | 0.071310 | 0.9930 | Success |
Overlapping template | 0.751572 | 0.9849 | Success |
Universal | 0.138860 | 0.9930 | Success |
Approximate entropy | 0.610070 | 0.9920 | Success |
Random excursions | 0.483876 | 0.9922 | Success |
Random excursions variant | 0.164773 | 0.9906 | Success |
Serial | 0.363593 | 0.9930 | Success |
Linear complexity | 0.088226 | 0.9850 | Success |
Table 1. Typical Result of NIST Statistical Tests
For the URNs (for instance
Figure 4.(a) Autocorrelation of uRNs (
From Fig.
Finally, the min-entropy evaluation procedure is proceed to estimate the randomness of output bit strings of the proposed UQRNG[
Through applying the min-entropy evaluation method mentioned previously, we respectively calculate the min-entropy of the output sequence generated from our system with ratios
Figure 5.Min-entropy evaluation.
We assume the fitted curve of min-entropy is
In conclusion, we demonstrated an experimental adjustable UQRNG which can generate accurate URNs of required proportion. The output BRNs can pass the 3-standard-deviation criteria and the NIST test suite. The proposed adjustable UQRNG overcomes the shortcomings of the first type of URNG based on BRNG, while producing true random URNs with approximately equal quality compared with that generated from the competing type of URNG. The generation rate of the proposed UQRNG can be increased by applying a light source of higher repetition frequency (1 GHz for example) or adjusting the attenuator to enhance the average photon numbers. However, at present the advanced commercial SPD has a maximum count rate of 100 MHz, which limits the final generation rate of the system. The Letter shows a proof of the proposed scheme. Research on other schemes to construct UQRNGs with a higher generation rate will be an emphasis of our work in the future. Another promising research direction in the future is searching for a method that can test the randomness of unbalanced random sequences.
References
[1] C. H. Bennett, G. Brassard. Proceedings of IEEE International Conference on Computers, Systems and Signal Processing, 175(1984).
[2] A. K. Ekert. Phys. Rev. Lett., 67, 661(1991).
[3] F. Tang, B. Zhu. Chin. Opt. Lett., 11, 090101(2013).
[4] W. Y. Hwang. Phys. Rev. Lett., 91, 057901(2003).
[5] H. K. Lo, H. F. Chau, M. Ardehali. J. Cryptol., 18, 133(2005).
[7] M. Isida, H. Ikeda. Ann. Inst. Stat. Math., 8, 119(1956).
[8] P. Xu, T. Wong, T. Horiuchi, P. Abshire. Electron. Lett., 42, 1346(2006).
[9] J. F. Dynes, Z. L. Yuan, A. L. Sharpe, A. J. Shields. Appl. Phys. Lett., 93, 031109(2008).
[10] A. Stefanov, N. Gisin, O. Guinnard, L. Guinnard, H. Zbinden. J. Mod. Opt., 47, 595(2000).
[12] Y. Q. Nie, H. F. Zhang, Z. Zhang, J. Wang, X. F. Ma. Appl. Phys. Lett., 104, 051110(2014).
[13] B. Qi, Y. Chi, H. K. Lo, Q. Li. Opt. Lett., 35, 312(2010).
[14] H. Guo, W. Tang, Y. Liu, W. Wei. Phys. Rev. E, 81, 051137(2010).
[16] P. Li, Y. C. Wang, J. Z. Zhang. Opt. Express, 18, 20360(2010).
[17] P. Li, Y. C. Wang, B. J. Wang. IEEE J. Sel. Top. Quantum Electron., 19, 0600208(2013).
[18] T. Lunghi, J. B. Brask, C. C. W. Lim, Q. Lavigne, J. Bowles, A. Martin, H. Zbinden, N. Brunner. A self-testing quantum random number generator(2014).
[19] G. Cañas, J. Cariñe, E. S. Gómez, J. F. Barra, A. Cabello, G. B. Xavier, G. Lima, M. Pawlowski. Experimental quantum randomness generation invulnerable to the detection loophole(2014).
[20] . NIST statistical test suite.
[21] X. Ma, F. Xu, H. Xu, X. Tan, B. Qi, H. K. Lo. Phys. Rev. A, 87, 062327(2013).
[22] F. Xu, B. Qi, X. Ma, H. Xu, H. Zheng, H. K. Lo. Opt. Express, 20, 12366(2012).
[23] A. B. Wang, P. Li, J. G. Zhang, J. Z. Zhang, L. Li, Y. C. Wang. Opt. Express, 21, 20452(2013).
[24] . ID Quantique system.
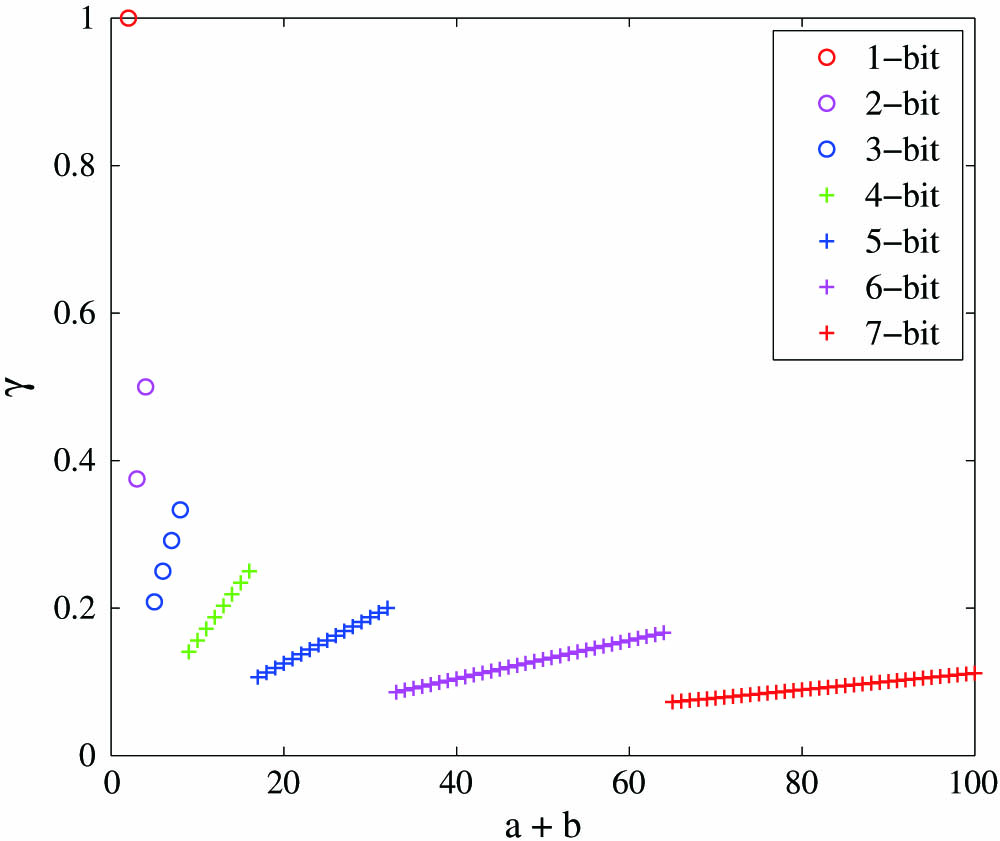
Set citation alerts for the article
Please enter your email address