Bendu BAI, Junpeng LI. Multi-exposure Image Fusion Based on Attention Mechanism[J]. Acta Photonica Sinica, 2022, 51(4): 0410004

Search by keywords or author
- Acta Photonica Sinica
- Vol. 51, Issue 4, 0410004 (2022)
Abstract
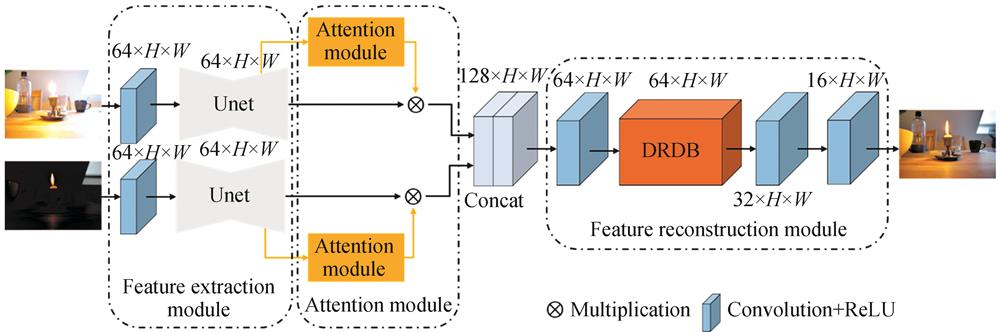
Set citation alerts for the article
Please enter your email address