Yongping HAO, Zhaorui CAO, Fan BAI, Haoyang SUN, Xing WANG, Jie QIN. Research on Infrared Visible Image Fusion and Target Recognition Algorithm Based on Region of Interest Mask Convolution Neural Network[J]. Acta Photonica Sinica, 2021, 50(2): 76

Search by keywords or author
- Acta Photonica Sinica
- Vol. 50, Issue 2, 76 (2021)
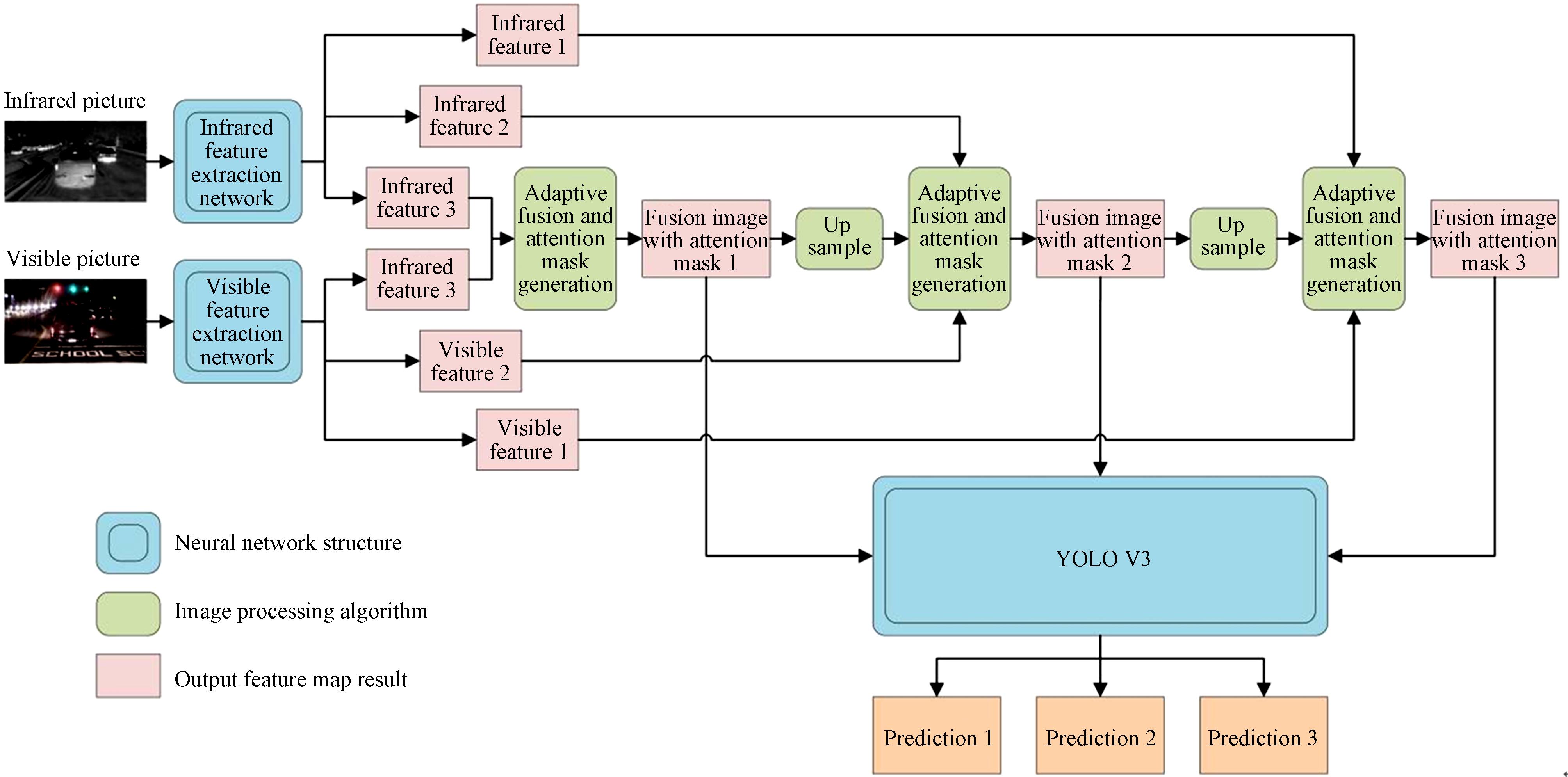
Fig. 1. Network structure of IVFNN
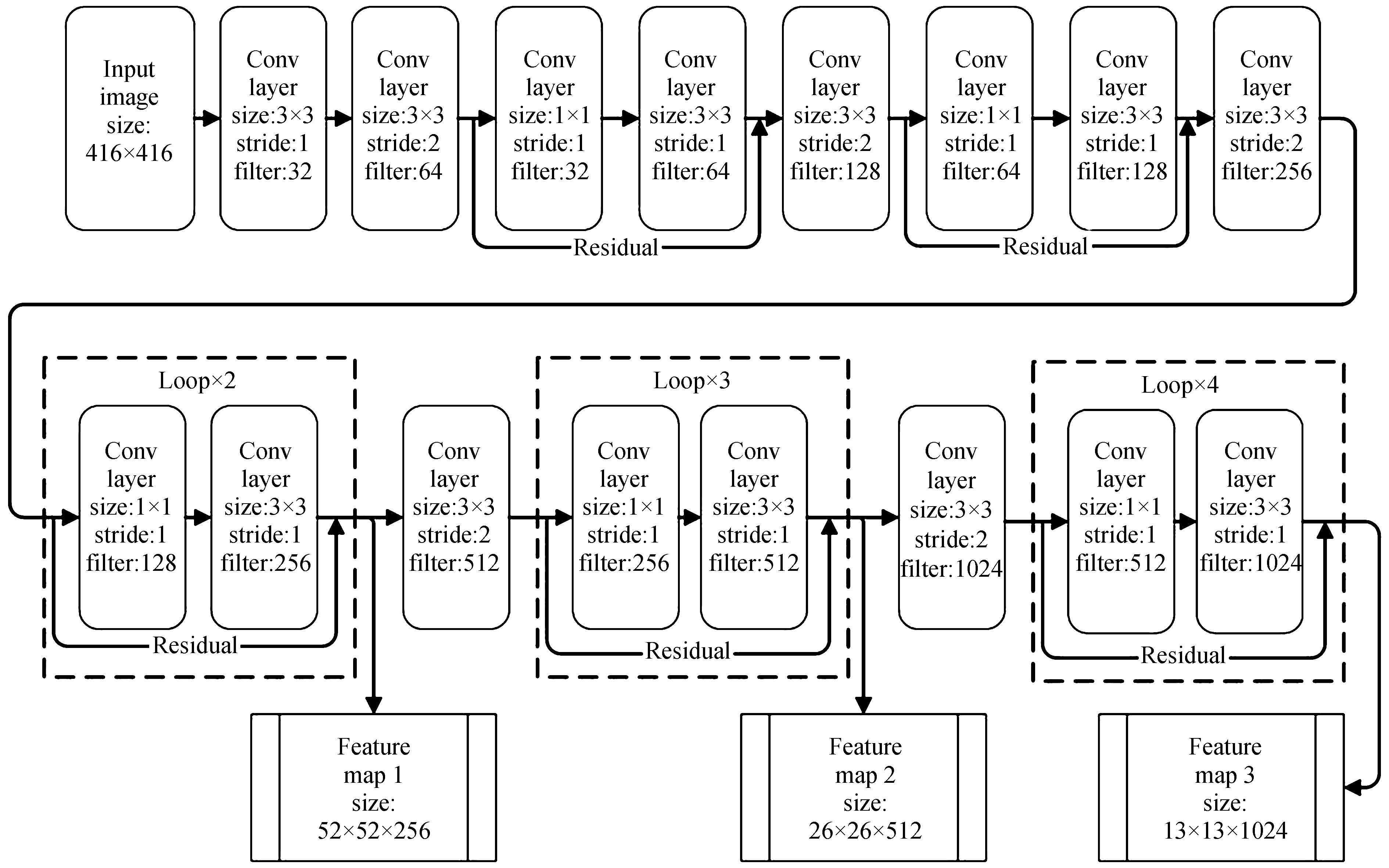
Fig. 2. Feature extraction network structure of IVFNN
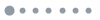
Fig. 3. Input image changes in the process of dual channel adaptive fusion and attention logic code calculation
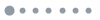
Fig. 4. Comparison of imaging contribution of infrared and visible light targets in different environments
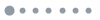
Fig. 5. Adaptive fusion process of infrared and visible feature maps group
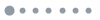
Fig. 6. Feature maps of each channel before and after fusion
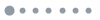
Fig. 7. The process of calculating the thermal radiation type type of image point
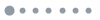
Fig. 8. Attention network in visible channel
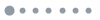
Fig. 9. Feature maps of each channel before and after adding the logical mask of ROI
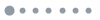
Fig. 10. Schematic diagram of CIOU in IVFNN
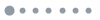
Fig. 11. Cross loss calculation of dual channels and fused images
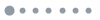
Fig. 12. Loss function curve of IVFNN
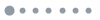
Fig. 13. Recognition effect of IVFNN and each frequency band
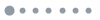
Fig. 14. Recognition effect of low-heat radiation target
|
Table 1. Multi-scale target recognition ability of IVFNN and each frequency band
|
Table 2. Comparison of test results of each algorithm
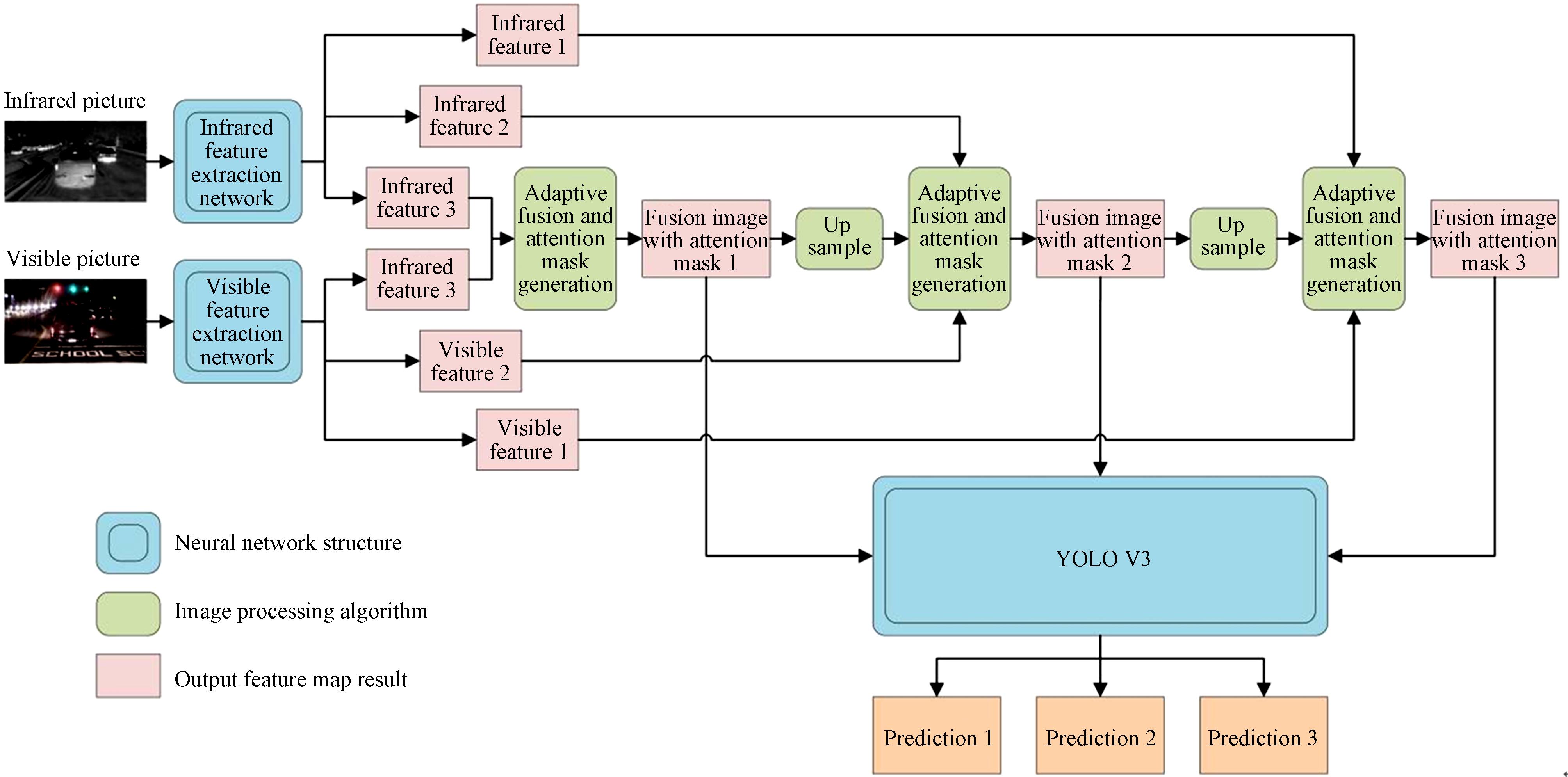
Set citation alerts for the article
Please enter your email address