ZHENG Zhi-qiang, LIU Yan-yan, PAN Chang-cheng, LI Guo-ning. Application of Improved YOLO V3 in Aircraft Recognition of Remote Sensing Images[J]. Electronics Optics & Control, 2019, 26(4): 28

Search by keywords or author
- Electronics Optics & Control
- Vol. 26, Issue 4, 28 (2019)
Abstract
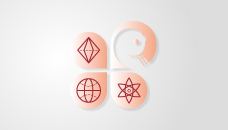
Set citation alerts for the article
Please enter your email address