SU Jiong, ZENG Zhigao, LIU Qiang, YI Shengqiu, WEN Zhiqiang, YUAN Xinpan. Optical Melanoma Image Detection Algorithm Based on Heavy Parameterized Large Kernel Convolution[J]. Semiconductor Optoelectronics, 2023, 44(5): 788

Search by keywords or author
- Semiconductor Optoelectronics
- Vol. 44, Issue 5, 788 (2023)
Abstract
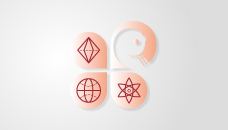
Set citation alerts for the article
Please enter your email address