LIU Yang, WANG Jun, WU Yunpeng1. WRSN charging path planning algorithm for improved Q-Learning[J]. Journal of Terahertz Science and Electronic Information Technology , 2022, 20(4): 393

Search by keywords or author
Journals >Journal of Terahertz Science and Electronic Information Technology >Volume 20 >Issue 4 >Page 393 > Article
- Journal of Terahertz Science and Electronic Information Technology
- Vol. 20, Issue 4, 393 (2022)
Abstract
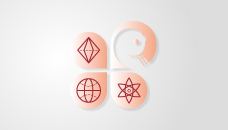
Set citation alerts for the article
Please enter your email address