Yuhui Huang, Xi’an Fan, Yanxi Zhang, Xiangdong Gao. Online Detection of Root Hump in Laser‐MIG Hybrid Welding Based on Invariable Moment Characteristics of Molten Pool Image[J]. Chinese Journal of Lasers, 2023, 50(12): 1202108

Search by keywords or author
- Chinese Journal of Lasers
- Vol. 50, Issue 12, 1202108 (2023)
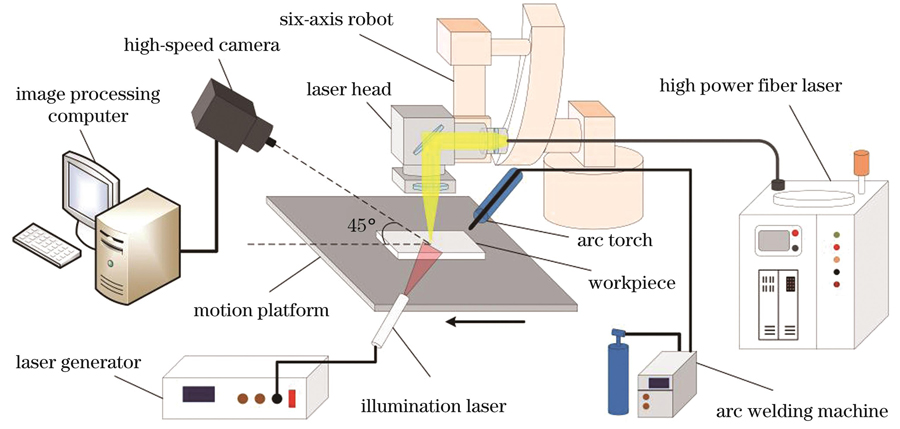
Fig. 1. Schematic of laser-MIG hybrid welding testing platform
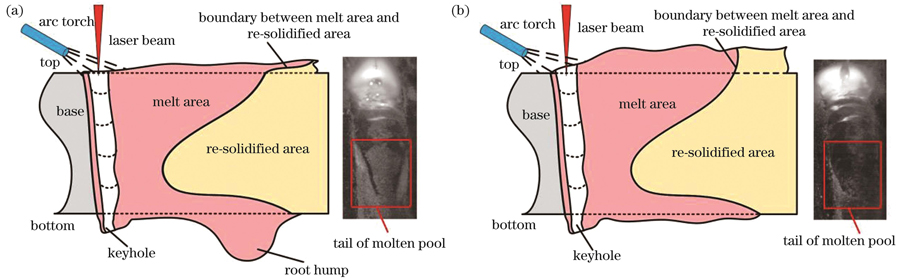
Fig. 2. Schematics of laser-MIG hybrid welding molten pool. (a) Formation process of root hump pool; (b) formation process of full penetration defect pool
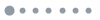
Fig. 3. Detection image in laser-MIG hybrid welding process
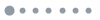
Fig. 4. Image processing of laser-MIG hybrid welding molten pool. (a) Original image; (b) molten pool image after MSR processing; (c) image of solidified molten pool; (d) image of molten pool tail
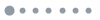
Fig. 5. Invariant moments and moving average values of molten pool tail images. (a) Invariant moment 1; (b) invariant moment 2; (c) invariant moment 3; (d) invariant moment 4
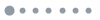
Fig. 6. Boxplots of invariant moment of molten pool tail image. (a) Invariant moment 1; (b) invariant moment 2; (c) invariant moment 3; (d) invariant moment 4
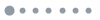
Fig. 7. One-dimensional convolutional neural network model for weld root hump detection
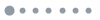
Fig. 8. Training results of one-dimensional convolutional neural network model for weld root hump detection. (a) Accuracy curves of model; (b) loss curves of model; (c) learning rate curve of model
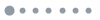
Fig. 9. Comparison of detection and real results of root hump
|
Table 1. Detection accuracies of model under different test samples
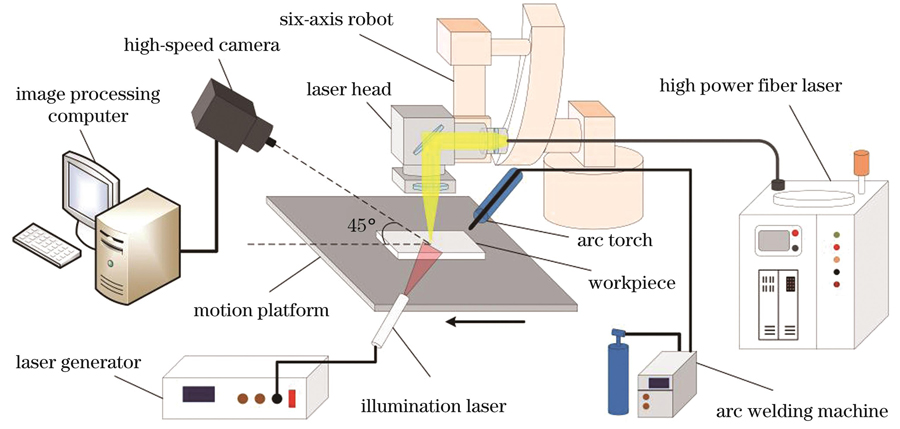
Set citation alerts for the article
Please enter your email address