SUN Guo-dong, ZHOU Zhen, WANG Jun-hao, ZHANG Yang, ZHAO Da-xing. Automatic fault recognition algorithm for key parts of train based on sparse coding based spatial pyramid matching and GA-SVM[J]. Optics and Precision Engineering, 2018, 26(12): 3087

Search by keywords or author
- Optics and Precision Engineering
- Vol. 26, Issue 12, 3087 (2018)
Abstract
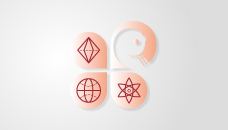
Set citation alerts for the article
Please enter your email address