WANG Lixiang, LIN Shanling, LIN Zhixian, GUO Tailiang. Image Target Detection System Based on Zynq Platform[J]. Semiconductor Optoelectronics, 2023, 44(1): 147

Search by keywords or author
- Semiconductor Optoelectronics
- Vol. 44, Issue 1, 147 (2023)
Abstract
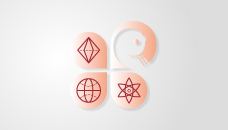
Set citation alerts for the article
Please enter your email address