Yihao Luo, Jun Zhang, Shiyin Du, Qiuquan Yan, Zeyu Zhao, Zilong Tao, Tong Zhou, Tian Jiang. Research Progress in Metamaterial Design and Fiber Beam Control Based on Deep Learning[J]. Chinese Journal of Lasers, 2023, 50(11): 1101007

Search by keywords or author
- Chinese Journal of Lasers
- Vol. 50, Issue 11, 1101007 (2023)
![Architecture of multi-layer perceptron network[29]. (a) Network structure; (b) mathematics process of perceptron](/richHtml/zgjg/2023/50/11/1101007/img_01.jpg)
Fig. 1. Architecture of multi-layer perceptron network[29]. (a) Network structure; (b) mathematics process of perceptron
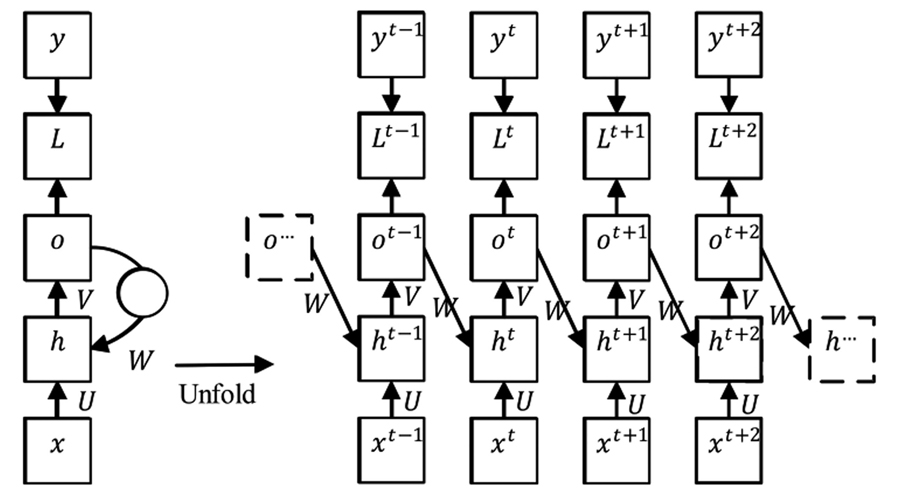
Fig. 2. Simple recurrent neural network and its computational graph
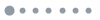
Fig. 3. Architecture of autoencoder
![Partition of material parameter space based on dielectric constant and permeability[5]](/Images/icon/loading.gif)
Fig. 4. Partition of material parameter space based on dielectric constant and permeability[5]
![Applications of metamaterials in various fields. (a) Optical communication[68]; (b) biomedicine[70]; (c) sensitive detection[69]](/Images/icon/loading.gif)
Fig. 5. Applications of metamaterials in various fields. (a) Optical communication[68]; (b) biomedicine[70]; (c) sensitive detection[69]
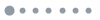
Fig. 6. Common metamaterial design methods
![Application of MLP in metamaterial design. (a) Using MLP to design nanoparticle[89]; (b) using MLP series to solve multi-solution problems[90]; (c) optimization and improvement of MLP—SMTL model[34]; (d) optimization and improvement of MLP—DEIFS algorithm[91]](/Images/icon/loading.gif)
Fig. 7. Application of MLP in metamaterial design. (a) Using MLP to design nanoparticle[89]; (b) using MLP series to solve multi-solution problems[90]; (c) optimization and improvement of MLP—SMTL model[34]; (d) optimization and improvement of MLP—DEIFS algorithm[91]
![Other deep learning methods for metamaterial design. (a) Using CNN to design binary imaged metasurface devices[92]; (b) using GAN to design metamaterial devices with complex topology[93]; (c) using DDQN to dynamically design device material types and structure parameters[94]; (d) using DEDGO to design a variety of low-dimensional heterostructures[95]; (e) using RNN to design metamaterial devices[18]](/Images/icon/loading.gif)
Fig. 8. Other deep learning methods for metamaterial design. (a) Using CNN to design binary imaged metasurface devices[92]; (b) using GAN to design metamaterial devices with complex topology[93]; (c) using DDQN to dynamically design device material types and structure parameters[94]; (d) using DEDGO to design a variety of low-dimensional heterostructures[95]; (e) using RNN to design metamaterial devices[18]
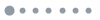
Fig. 9. Basic model of intelligent optimization algorithm in optical system
![Intelligent mode-locked laser structures based on different search methods. (a) Experimental structure of self-optimizing mode-locked laser based on genetic algorithm[112]; (b) experimental structure of intelligent mode-locked fiber laser based on HLA algorithm[113]](/Images/icon/loading.gif)
Fig. 10. Intelligent mode-locked laser structures based on different search methods. (a) Experimental structure of self-optimizing mode-locked laser based on genetic algorithm[112]; (b) experimental structure of intelligent mode-locked fiber laser based on HLA algorithm[113]
![Intelligent mode-locked laser structures based on different deep reinforcement learning algorithms. (a) Adaptive mode-locked laser model designed using DQN algorithm[115]; (b) automatic mode-locked laser system based on DELAY algorithm[24]; (c) mode-locked laser system based on reinforcement learning and spectral learning[116]; (d) intelligent mode-locked laser architecture based on TD3 algorithm[117]](/Images/icon/loading.gif)
Fig. 11. Intelligent mode-locked laser structures based on different deep reinforcement learning algorithms. (a) Adaptive mode-locked laser model designed using DQN algorithm[115]; (b) automatic mode-locked laser system based on DELAY algorithm[24]; (c) mode-locked laser system based on reinforcement learning and spectral learning[116]; (d) intelligent mode-locked laser architecture based on TD3 algorithm[117]
![Control structure of partially coherent optical systems. (a) Coherent beam combination system for 4-beam fiber amplifier based on SPGD algorithm[119]; (b) two-stage phase control system based on deep learning model and SPGD algorithm[120]](/Images/icon/loading.gif)
Fig. 12. Control structure of partially coherent optical systems. (a) Coherent beam combination system for 4-beam fiber amplifier based on SPGD algorithm[119]; (b) two-stage phase control system based on deep learning model and SPGD algorithm[120]
![Coherent optical neuron system[123]](/Images/icon/loading.gif)
Fig. 13. Coherent optical neuron system[123]
|
Table 1. Comparison of different algorithms in mode-locking time
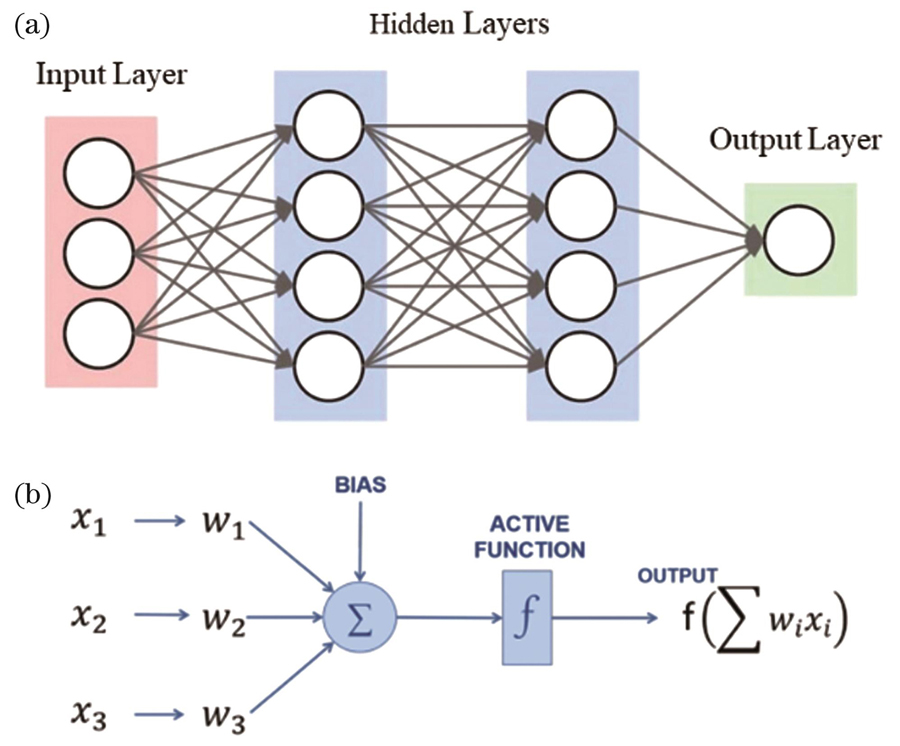
Set citation alerts for the article
Please enter your email address