Ang CHEN, Xiuchun YANG, Bin XU, Yunxiang JIN, Wenbo ZHANG, Jian GUO, Xiaoyu XING, Dong YANG. Research on Recognition Methods of Elm Sparse Forest based on Object-based Image Analysis and Deep Learning [J]. Journal of Geo-information Science, 2020, 22(9): 1897

Search by keywords or author
- Journal of Geo-information Science
- Vol. 22, Issue 9, 1897 (2020)
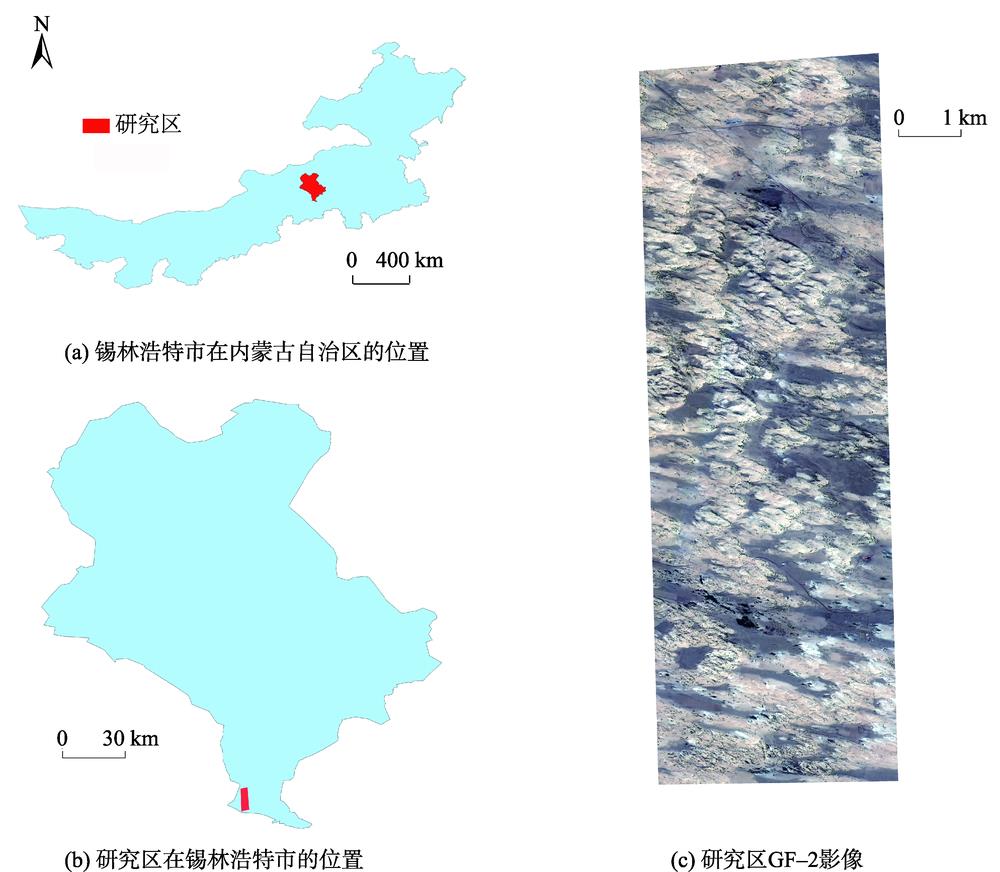
Fig. 1. Location map of study area in Hunshandake sandy land
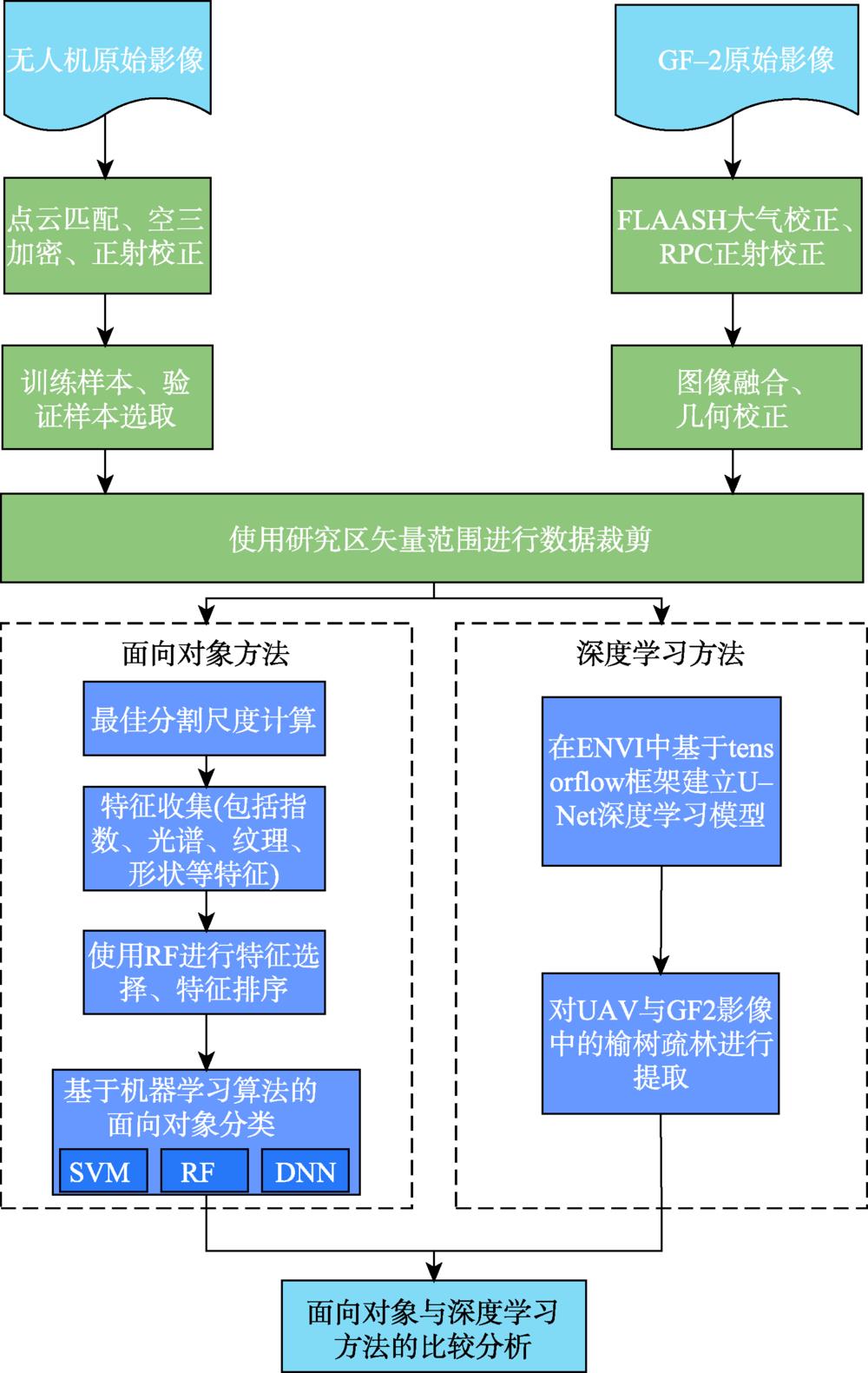
Fig. 2. Flow chart of research methods
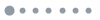
Fig. 3. Deep learning training samples
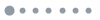
Fig. 4. LV and LV-ROC Curves of UAV Image
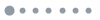
Fig. 5. Optimum segmentation of elm sparse forest in UAV image
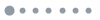
Fig. 6. LV and LV-ROC Curves of GF-2 Image
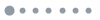
Fig. 7. Optimum segmentation of elm sparse forest in GF-2 image
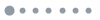
Fig. 8. Sequencing of the Importance of UAV image features
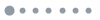
Fig. 9. Sequencing of the Importance of GF-2image features
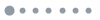
Fig. 10. Accuracy comparison of SVM、RF、DNN used in OBIA
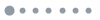
Fig. 11. Accuracy comparison between object-based method and deep learning method
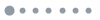
Fig. 12. Comparison of extraction result between OBIA and deep learning for elm sparse forest
|
Table 1. UAV flight parameters
|
Table 2. Feature list in OBIA
|
Table 3. Results of GF-2 image using deep learning method
|
Table 4. Results of UAV image using deep learning method
Set citation alerts for the article
Please enter your email address