Jupu YANG, Jialin DU, Fanxing LI, Qingrong CHEN, Simo WANG, Wei YAN. Deep Learning Based Method for Automatic Focus Detection in Digital Lithography[J]. Acta Photonica Sinica, 2022, 51(6): 0611002

Search by keywords or author
- Acta Photonica Sinica
- Vol. 51, Issue 6, 0611002 (2022)
Abstract
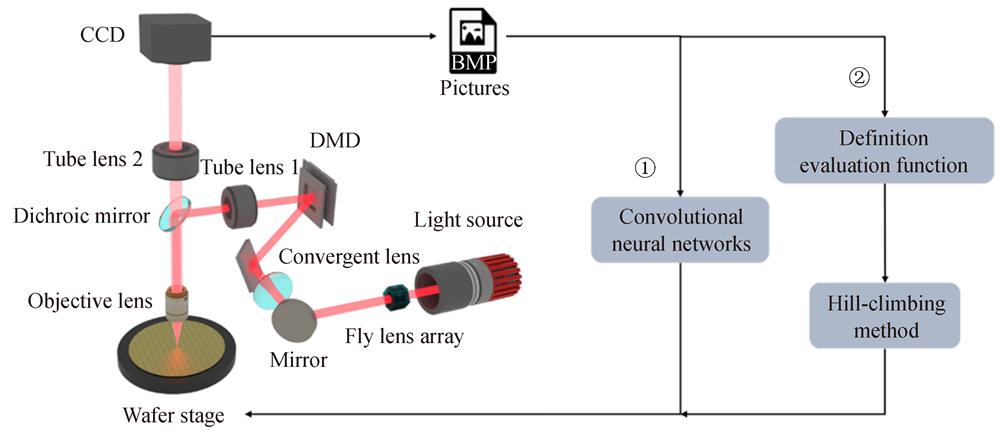
Set citation alerts for the article
Please enter your email address