The connection between Maxwell’s equations and neural networks opens unprecedented opportunities at the interface between photonics and deep learning. This feature issue highlights recent research progress at the interdisciplinary field of photonics and deep learning and provides an opportunity for different communities to exchange their ideas from different perspectives.

- Photonics Research
- Vol. 9, Issue 8, DLP1 (2021)
Abstract
The application of deep learning in photonics has gained a tremendous amount of attention in the past few years. This interdisciplinary research covers a broad range of topics, including the inverse design of photonic devices, enhanced sensing and imaging, neuromorphic computing, and many other emerging applications. This feature issue provides a snapshot of current research with a collection of recent advances in this thriving field. We feature 28 papers contributed by experts from various aspects of photonics research.
The first collection of works in this issue focuses on using deep learning for device design. Conventional design of photonic structures often requires solving Maxwell’s equations on a large scale. However, our capability to solve Maxwell’s equation has significantly lagged behind today’s fabrication capability that routinely produces devices with millions of nanostructure features. Using neural networks for photonic design allows researchers to tap into a rich set of machine-learning algorithms which enable fast inverse design and optimization. Examples include on-demand design of spectrally sensitive multi-band absorber [1], Fabry–Pérot-cavity-based color filters far beyond sRGB [2], transmitted metasurface cloak by tandem neural network [3] and intelligent coding metasurface hologram by physics assisted unsupervised generative adversarial network [4]. Strategy that combines deep neural network with genetic algorithms requires significantly less training data and enables highly efficient inverse designs [5]. For a more comprehensive review of past research and future perspective, Yongmin Liu and Peter R. Wiecha are invited to contribute two reviews on how to interface photonics with artificial intelligence, with focus on the inverse design strategy and applications beyond inverse design [6,7].
Deep learning could also help to deepen our understanding of complex nanophotonic structures. Emerging complex photonic structures derive their properties from a large network of inter-dependent nano-elements with both local and global connections. The vast parameter space offers unprecedented opportunities for device application, but at the same time presents a daunting challenge for developing an understanding of such complex structures. Deep learning could leverage such problems to recognize misaligned hyperfine orbital angular momentum modes [8], achieve phase compensation for free-space angular momentum-encoded quantum key distribution [9], enable automatic highly efficient photon distribution estimation via deep reinforcement learning [10], engineer multiple bound states in the continuum by latent representation of freeform structures [11], and identify modulation format in fiber communications using a single dynamical node [12].
Sign up for Photonics Research TOC. Get the latest issue of Photonics Research delivered right to you!Sign up now
The ever-increasing computing power required by deep learning prompts the search for alternative computing methods that are faster and more energy efficient. Optical analog computing can be passive with minimal energy consumption, and more importantly, its intrinsic parallelism can significantly accelerate computing speed. Such a capability is potentially useful as an optical preprocessor for real-time high-throughput image processing. Wave dynamics in a highly scattering medium can provide an alternative way to perform neuromorphic computing. Nanostructured photonic devices can exploit sub-wavelength linear and nonlinear scatterers to realize complex input-output mapping far beyond the capabilities of traditional nanophotonic devices. Theoretical and experimental exploration works in this feature issue have discussed neuromorphic node based on quantum dot laser [13], end-to-end optical backpropagation for training neural networks [14], free-space optical neural network based on thermal atomic nonlinearity [15], deep-learning empowered dynamic wavefront shaping in nonstationary scattering media [16], delay weight plasticity based on supervised learning in photonic spiking neural networks [17], and all-optical neuromorphic binary convolution with spiking VCSEL neurons [18].
Another area of great interest is the application of deep learning in imaging analysis and computational cameras. Representative examples include deep compressive imaging techniques via optimized-pattern scanning [19], compressed ultrafast photography via an augmented-Lagrangian and deep-learning hybrid algorithm [20], and deep plug-and-play priors for spectral snapshot compressive imaging [21]. Image reconstruction techniques include denoising and reconstruction of super-resolution structured illumination microscopy images [22], and a simple low-SWaP hybrid machine vision system for universal training and generalized image reconstruction [23]. Novel imaging strategies through unknown scattering media based on physics informed learning [24], and incoherent imaging through highly dynamic and optically thick turbid media [25] are also demonstrated.
The last area that this feature issue highlights is the use of deep learning for sensors such as a smart ring resonator-based sensor for multicomponent chemical analysis [26] and sensing in the presence of strong noise by deep learning of dynamic multimode fiber interference [27]. A real-time deep learning design tool for far-field radiation profile [28] has been demonstrated for easy adoption and accessibility for device designers.
We take this opportunity to thank Prof. Lan Yang, the Editor-in-Chief, and Prof. Cun-Zheng Ning, the Deputy Editor for inviting us to organize this feature issue. They provide many helpful suggestions that greatly improve this feature issue. We also want to thank the editorial staff for their great support. We are also grateful to the reviewers that have helped us assess the quality and originality of the large amount of submitted papers, while keeping a high standard for our feature issue. Finally, and most importantly, we thank all the authors for submitting and contributing to this feature issue which provides a snapshot of exciting ongoing research work in the field and the opportunities that deep learning tools offer for the future of photonics technologies.
References
[1] S. So, Y. Yang, T. Lee, J. Rho. On-demand design of spectrally sensitive multiband absorbers using an artificial neural network. Photon. Res., 9, B153-B158(2021).
[2] P. Dai, Y. Wang, Y. Hu, C. H. de Groot, O. Muskens, H. Duan, R. Huang. Accurate inverse design of Fabry–Pérot-cavity-based color filters far beyond sRGB via a bidirectional artificial neural network. Photon. Res., 9, B236-B246(2021).
[3] Z. Zhen, C. Qian, Y. Jia, Z. Fan, R. Hao, T. Cai, B. Zheng, H. Chen, E. Li. Realizing transmitted metasurface cloak by a tandem neural network. Photon. Res., 9, B229-B235(2021).
[4] C. Liu, W. M. Yu, Q. Ma, L. Li, T. J. Cui. Intelligent coding metasurface holograms by physics-assisted unsupervised generative adversarial network. Photon. Res., 9, B159-B167(2021).
[5] Y. Ren, L. Zhang, W. Wang, X. Wang, Y. Lei, Y. Xue, X. Sun, W. Zhang. "Genetic-algorithm-based deep neural networks for highly efficient photonic device design. Photon. Res., 9, B247-B252(2021).
[6] Y. Xu, X. Zhang, Y. Fu, Y. Liu. Interfacing photonics with artificial intelligence: an innovative design strategy for photonic structures and devices based on artificial neural networks. Photon. Res., 9, B135-B152(2021).
[7] P. R. Wiecha, A. Arbouet, C. Girard, O. L. Muskens. Deep learning in nano-photonics: inverse design and beyond. Photon. Res., 9, B182-B200(2021).
[8] X. Wang, Y. Qian, J. J. Zhang, G. Ma, S. Zhao, R. F. Liu, H. Li, P. Zhang, H. Gao, F. Huang, F. Li. Learning to recognize misaligned hyperfine orbital angular momentum modes. Photon. Res., 9, B81-B86(2021).
[9] X. Wang, T. Wu, C. Dong, H. Zhu, Z. Zhu, S. Zhao. Integrating deep learning to achieve phase compensation for free-space orbital-angular-momentum-encoded quantum key distribution under atmospheric turbulence. Photon. Res., 9, B9-B17(2021).
[10] J. Ma, Z. Piao, S. Huang, X. Duan, G. Qin, L. Zhou, Y. Xu. Monte Carlo simulation fused with target distribution modeling via deep reinforcement learning for automatic high-efficiency photon distribution estimation. Photon. Res., 9, B45-B56(2021).
[11] R. Lin, Z. Alnakhli, X. Li. Engineering of multiple bound states in the continuum by latent representation of freeform structures. Photon. Res., 9, B96-B103(2021).
[12] Q. Cai, Y. Guo, P. Li, A. Bogris, K. A. Shore, Y. Zhang, Y. Wang. Modulation format identification in fiber communications using single dynamical node-based photonic reservoir computing. Photon. Res., 9, B1-B8(2021).
[13] G. Sarantoglou, M. Skontranis, A. Bogris, C. Mesaritakis. Experimental study of neuromorphic node based on a multiwaveband emitting two-section quantum dot laser. Photon. Res., 9, B87-B95(2021).
[14] X. Guo, T. D. Barrett, Z. M. Wang, A. I. Lvovsky. Backpropagation through nonlinear units for the all-optical training of neural networks. Photon. Res., 9, B71-B80(2021).
[15] A. Ryou, J. Whitehead, M. Zhelyeznyakov, P. Anderson, C. Keskin, M. Bajcsy, A. Majumdar. Free-space optical neural network based on thermal atomic nonlinearity. Photon. Res., 9, B128-B134(2021).
[16] Y. Luo, S. Yan, H. Li, P. Lai, Y. Zheng. Towards smart optical focusing: deep learning empowered dynamic wavefront shaping through nonstationary scattering media. Photon. Res., 9, B262-B278(2021).
[17] Y. Han, S. Xiang, Z. Ren, C. Fu, A. Wen, Y. Hao. Delay-weight plasticity-based supervised learning in optical spiking neural networks. Photon. Res., 9, B119-B127(2021).
[18] Y. Zhang, J. Robertson, S. Xiang, M. Hejda, J. Bueno, A. Hurtado. All-optical neuromorphic binary convolution with a spiking VCSEL neuron for image gradient magnitudes. Photon. Res., 9, B201-B209(2021).
[19] K. Zhang, J. Hu, W. Yang. Deep compressed imaging via optimized pattern scanning. Photon. Res., 9, B57-B70(2021).
[20] C. Yang, Y. Yao, C. Jin, D. Qi, F. Cao, Y. He, J. Yao, P. Ding, L. Gao, T. G. Jia, J. Liang, Z. Sun, S. Zhang. High-fidelity image reconstruction for compressed ultrafast photography via an augmented-Lagrangian and deep-learning hybrid algorithm. Photon. Res., 9, B30-B37(2021).
[21] S. Zheng, Y. Liu, Z. Meng, M. Qiao, Z. Tong, X. Yang, S. Han, X. Yuan. Deep plug-and-play priors for spectral snapshot compressive imaging. Photon. Res., 9, B18-B29(2021).
[22] Z. H. Shah, M. Müller, T.-C. Wang, P. M. Scheidig, A. Schneider, M. Schüttpelz, T. Huser, W. Schenck. Deep-learning based denoising and reconstruction of super-resolution structured illumination microscopy images. Photon. Res., 9, B168-B181(2021).
[23] B. Muminov, A. Perry, R. Hyder, M. S. Asif, L. T. Vuong. Toward simple, generalizable neural networks with universal training for low-SWaP hybrid vision. Photon. Res., 9, B253-B261(2021).
[24] S. Zhu, E. Guo, J. Gu, L. Bai, J. Han. Imaging through unknown scattering media based on physics-informed learning. Photon. Res., 9, B210-B219(2021).
[25] S. Zheng, H. Wang, S. Dong, F. Wang, G. Situ. Incoherent imaging through highly nonstatic and optically thick turbid media based on neural network. Photon. Res., 9, B220-B228(2021).
[26] Z. Li, H. Zhang, B. T. T. Nguyen, S. Luo, P. Y. Liu, J. Zou, Y. Shi, H. Cai, Z. Yang, Y. Jin, Y. Hao, Y. Zhang, A.-Q. Liu. Smart ring resonator–based sensor for multicomponent chemical analysis via machine learning. Photon. Res., 9, B38-B44(2021).
[27] L. V. Nguyen, C. C. Nguyen, G. Carneiro, H. Ebendorff-Heidepriem, S. C. Warren-Smith. Sensing in the presence of strong noise by deep learning of dynamic multimode fiber interference. Photon. Res., 9, B109-B118(2021).
[28] J. Qie, E. Khoram, D. Liu, M. Zhou, L. Gao. Real-time deep learning design tool for far-field radiation profile. Photon. Res., 9, B104-B108(2021).
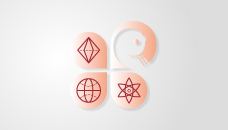
Set citation alerts for the article
Please enter your email address