LIAO Yi-peng, ZHANG Jin, WANG Zhi-gang, WANG Wei-xing. Flotation performance recognition based on dual-modality multiscale CNN features and adaptive deep learning KELM[J]. Optics and Precision Engineering, 2020, 28(8): 1785

Search by keywords or author
- Optics and Precision Engineering
- Vol. 28, Issue 8, 1785 (2020)
Abstract
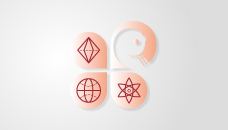
Set citation alerts for the article
Please enter your email address