
- Advanced Photonics
- Vol. 5, Issue 2, 020501 (2023)
Abstract
Machine learning (ML) is one of the most acclaimed areas of science and technology, leading to countless real-world benefits, from industry to healthcare. ML involves creating algorithms to perform specific tasks by recognizing patterns in the data and without requiring extensive instructions, namely without being explicitly programmed. This possibility has recently attracted the attention of a broad community of scientists interested in learning, controlling, and making optimal use of quantum systems of increasing complexity,1,2 potentially leading to revolutionary progresses in many fields.
Quantum metrology is among the most promising branches of quantum-enhanced technology.3,4 It studies the design and engineering of quantum probes, noise resilient evolution and optimal measurements, in order to enhance the estimation of physical parameters beyond the current state-of-the-art. Applications in optics5 include imaging, biological sensing, interferometry and gravitational wave detection. In realistic scenarios, resources (e.g., time, energy, number of particles, etc.) are always limited. These constraints pose important challenges in quantum metrology that can be successfully faced by taking advantage of the ML techniques and ideas developed in recent years.
First, every quantum metrology device needs to be calibrated. It is crucial to understand how the statistics of the measurement outcomes depend on the parameters of interest. It has been shown that a deep neural network trained with a relatively modest amount of calibration data is able to capture very efficiently the connection between measurement results and parameter values.6
Sign up for Advanced Photonics TOC. Get the latest issue of Advanced Photonics delivered right to you!Sign up now
As reported recently in Advanced Photonics, Cimini et al.17 introduce a black-box approach to optical multiphase estimation where the experimental device is assisted by an ML agent only processing bare data (see schematic in Fig. 1). The information about the phases to be estimated is continuously updated, depending on the Bayesian distribution that a trained deep neural network associates to each measurement event.9 Furthermore, reinforcement learning is used to find an efficient experiment-design heuristic for Bayesian estimation15 in order to optimize the experimental setting (here in the form of adjustable phase shifts), based on the current knowledge of the parameter and available resources. The combination of the two techniques is an example of deep reinforcement learning1,2 applied here, for the first time, to optical quantum metrology. The method achieves better performances than more conventional techniques not based on ML. It is, finally, important to emphasize that the complexity of multiparameter estimation raises quickly with the number of unknown parameters, and advanced numerical methods such as those demonstrated by Cimini et al. are necessary to efficiently handle challenging applications in imaging and sensing.
Figure 1.A general quantum metrology device consists of quantum state preparation, parameter-encoding evolution and measurement. Here, the device is equipped with an ML agent. The agent associates to each measurement result
References
[1] V. Gebhart et al. Learning quantum systems. Nat. Rev. Phys.(2023).
[2] A. Dawid et al. Modern applications of machine learning in quantum science(2022).
[5] E. Polino et al. Photonic quantum metrology. AVS Quantum Sci., 2, 024703(2020).
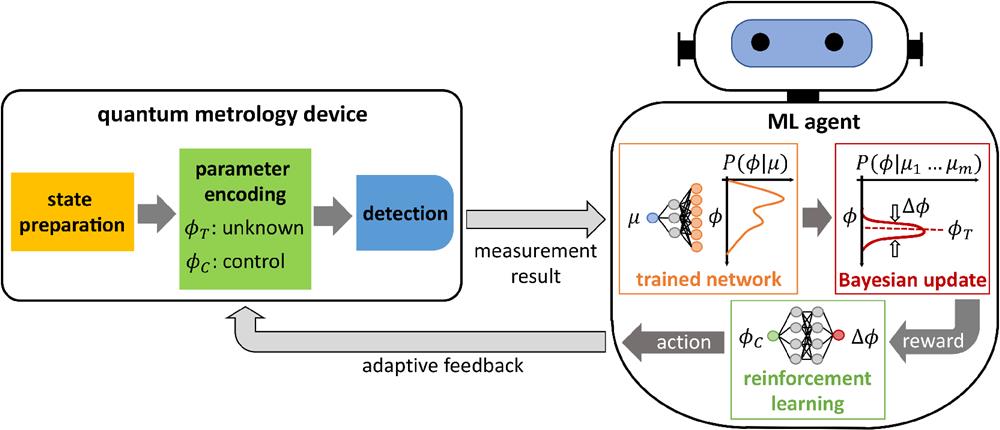
Set citation alerts for the article
Please enter your email address