Huijuan TIAN, Jiahao ZHAI, Jianxin LIU, Jiawei LIU, Linlin DENG. A Low-quality Iris Image Segmentation Algorithm Based on SRN-UNet[J]. Acta Photonica Sinica, 2022, 51(2): 0210006

Search by keywords or author
- Acta Photonica Sinica
- Vol. 51, Issue 2, 0210006 (2022)
Abstract
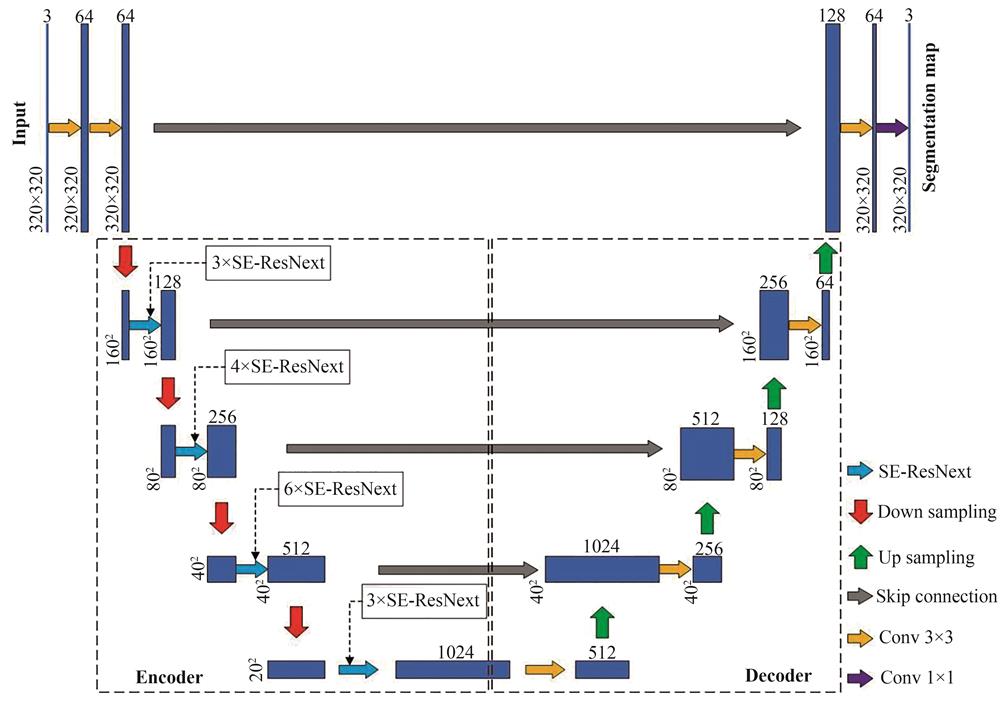
Set citation alerts for the article
Please enter your email address