Haicheng QU, Lei SHEN. SAR Image Denoising and Semantic Enhancement for Object Detection[J]. Acta Photonica Sinica, 2022, 51(4): 0410003

Search by keywords or author
- Acta Photonica Sinica
- Vol. 51, Issue 4, 0410003 (2022)
Abstract
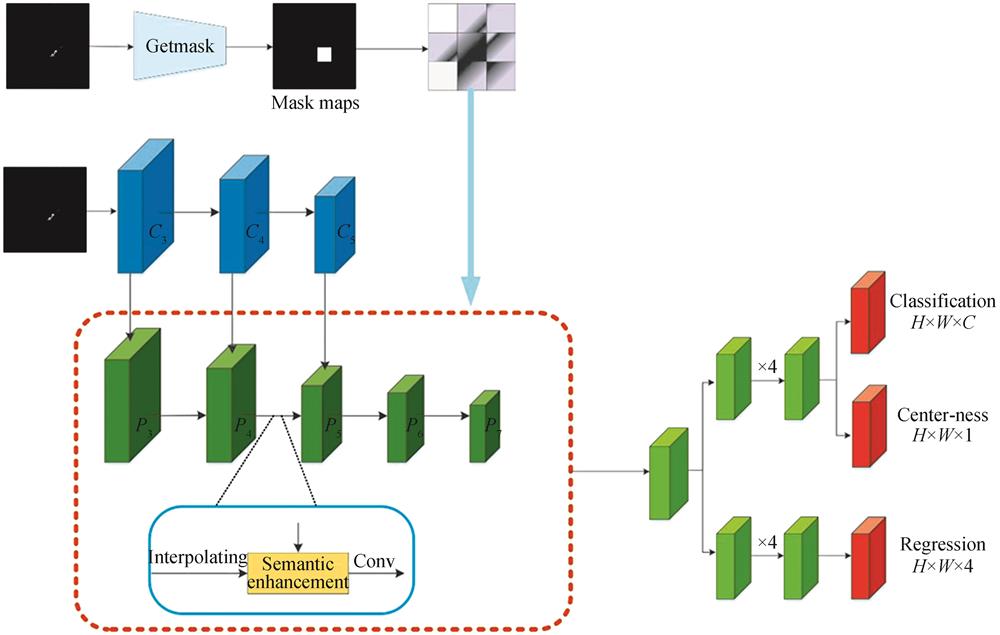
Set citation alerts for the article
Please enter your email address