Chenjin Deng, Long Pan, Chenglong Wang, Xin Gao, Wenlin Gong, Shensheng Han, "Performance analysis of ghost imaging lidar in background light environment," Photonics Res. 5, 431 (2017)

Search by keywords or author
- Photonics Research
- Vol. 5, Issue 5, 431 (2017)
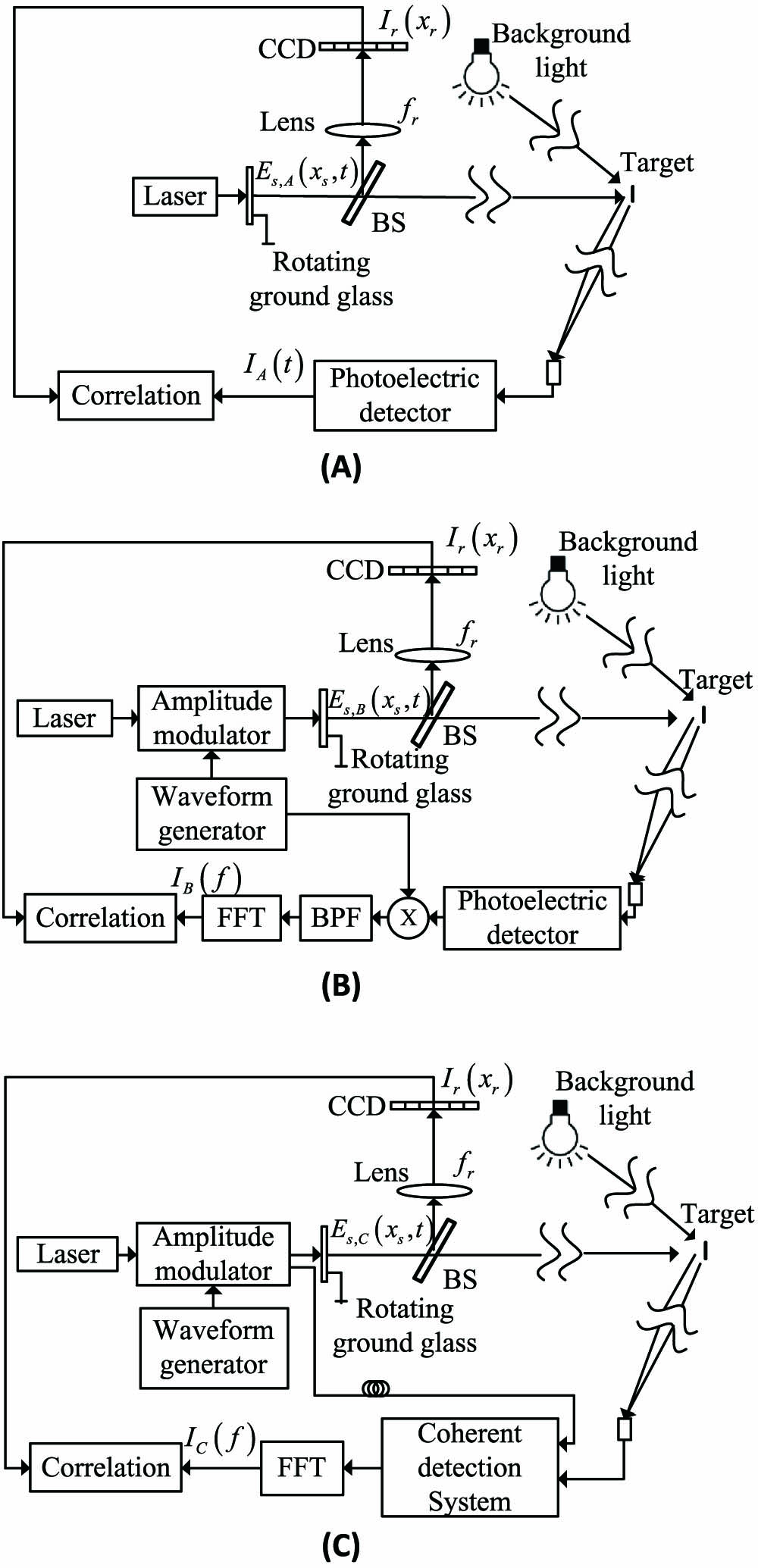
Set citation alerts for the article
Please enter your email address