Author Affiliations
1Zhejiang University, ZJU-UIUC Institute, Interdisciplinary Center for Quantum Information, State Key Laboratory of Extreme Photonics and Instrumentation, Hangzhou, China2Zhejiang University, ZJU-Hangzhou Global Science and Technology Innovation Center, Key Laboratory of Advanced Micro/Nano Electronic Devices and Smart Systems of Zhejiang, Hangzhou, China3Zhejiang University, Jinhua Institute of Zhejiang University, Jinhua, Chinashow less

Fig. 1. Schematic of autonomous aeroamphibious invisibility cloak. The invisible drone is integrated with perception, decision, and action modules to allow it to self-adapt to kaleidoscopic environments and offset external detection without human intervention. The perception module mainly includes a custom-built EM detector for capturing incoming waves, a gyroscope for sensing attitude, acceleration speed, and angular velocity, and a camera for obtaining the surrounding environment. The detected information, together with user-defined cloaking pictures, is input into a pretrained deep-learning model to instruct the drone to make action at a millisecond scale. According to the output, the reconfigurable spatiotemporal metasurface veneers globally manipulate the scattering wave by directly controlling the temporal sequence of each meta-atom. As a consequence, when freely shuttling among sea, land, and air, the drone can maintain invisibility at all times or disguise itself into other illusive scattering appearances. Such an aeroamphibious cloak constitutes a big milestone to assist conventional proof-of-concept metamaterials-based invisibility cloaks to go out of laboratories.

Fig. 2. Design and working mechanism of spatiotemporal metasurfaces. (a) The spatiotemporal metasurfaces are composed of an array of reconfigurable meta-atoms at microwave, each of which incorporates two PIN diodes. The specific geometries of metasurfaces are located in Supplementary Note 1 in the Supplementary Material. By feeding periodic time-varying voltage sequences, the spatiotemporal metasurfaces generate a series of reflected harmonic waves with customized scattering pattern and power distribution. (b) Reflection response of metasurfaces by applying different bias voltages across the loaded diodes. Two diodes correspond to four reflected states. At 3.1 GHz, the reflected phase has a uniform interval, while the reflected amplitude keeps high. (c) Synthetic reflection states at center frequency. By controlling the time-varying sequences (the period is 8) of the meta-atom, a constellation of equivalent reflection states is synthesized to occupy the complex plane. Here we want to underscore that one time-varying series can only induce one equivalent state; however, one equivalent state can be induced by more-than-one time-varying series. (d) Comparison between spatial and spatiotemporal metasurfaces. For a given three-dimensional scattering pattern, the spatiotemporal modulation allows a high degree of freedom to mimic the ground truth with the SSIM of 94.03%, in contrast to 88.64% by spatial-only modulation (with only four initial reflected states). Here conventional GA is adopted to optimize the profiles of metasurfaces. SSIM, structural similarity and GA, genetic algorithm.
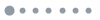
Fig. 3. Architecture of stochastic-evolution learning that drives the autonomous invisible drone. (a) The proposed network consists of two cascaded networks, namely, the generation network and the elimination network. The CVAE-based generation network, composed of a recognition module, a latent space, and a reconstruction module, is used to produce diverse candidates, and the elimination network, a fully connected neural network, is launched to filter all inferior candidates. The layer-level illustration of the network and complete training process are given in Supplementary Note 5 in the Supplementary Material. The 10 sets of Gaussian variational parameters generated from the preceding layer of the recognition module constitute the latent space. (b) Latent space visualization. For each point in the extracted Gaussian distribution, the output metasurface distribution is retrieved by concatenating the sampled point (i.e., latent variable) with the far-field pattern (i.e., label information) and then going through the reconstruction module. (c) Test instances. The deep-learning prediction is filtered by the elimination network to retain the best one. One prominent advantage is that such a framework can effectively address the nonuniqueness issue in inverse design and provide users with more than one answer.
![Experimental measurement of autonomous invisible drone flying in the sky. (a) Experimental setup of the intelligent invisible drone outside the laboratory. The invisible drone freely flies in the sky and passes through a conical detection region excited by a transmitting antenna, during which three antennas detect the scattering waves in real time. The dotted curve shows the flight trajectory. VNA, vector network analyzer. (b) Photograph of intelligent invisible drone. (c), (d) Simulation results when the cloaked/bare drone is impinged by an obliquely incident wave. Evidently, the bare drone produces strong scattering field that exposes it to foe radar, while the cloaked drone largely absorbs the incident wave. (e) Experimental time-varying electric field by the three receivers. Interestingly, the signal remains almost stable and matches with the background when the cloaked drone flies from the left to the right, in stark contrast to the erratic fluctuation in the uncloaked case (Video 1, mp4, 28.9 MB [URL: https://doi.org/10.1117/1.AP.6.1.016001.s1]; Video 2, mp4, 22.4 MB [URL: https://doi.org/10.1117/1.AP.6.1.016001.s2]).](/Images/icon/loading.gif)
Fig. 4. Experimental measurement of autonomous invisible drone flying in the sky. (a) Experimental setup of the intelligent invisible drone outside the laboratory. The invisible drone freely flies in the sky and passes through a conical detection region excited by a transmitting antenna, during which three antennas detect the scattering waves in real time. The dotted curve shows the flight trajectory. VNA, vector network analyzer. (b) Photograph of intelligent invisible drone. (c), (d) Simulation results when the cloaked/bare drone is impinged by an obliquely incident wave. Evidently, the bare drone produces strong scattering field that exposes it to foe radar, while the cloaked drone largely absorbs the incident wave. (e) Experimental time-varying electric field by the three receivers. Interestingly, the signal remains almost stable and matches with the background when the cloaked drone flies from the left to the right, in stark contrast to the erratic fluctuation in the uncloaked case (Video 1, mp4, 28.9 MB [URL: https://doi.org/10.1117/1.AP.6.1.016001.s1]; Video 2, mp4, 22.4 MB [URL: https://doi.org/10.1117/1.AP.6.1.016001.s2]).
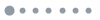
Fig. 5. Experimental demonstration of autonomous invisible drone amidst amphibious background. (a) Schematic illustration of the intelligent invisible drone when it lands on grassland. Eight receiving antennas are randomly distributed along the arc to detect the surrounding scattered wave. The right insets show different scenes, including sand and sea. is the tilt angle of the drone. Scenes 4 and 5 show the illusive purpose enabled by the invisible drone that reconfigures itself as a giraffe and a shark. (b) Experimental results of a cloaked drone, in comparison with the pure background and bare drone. The relative height of the colored circle represents the electric field strength. The higher the circle location is, the larger the -field strength is. Here the results are quantified with the Pearson correlation coefficient to depict the similarity between the background and the cloaked/bare drone. The cloaked drone is well blended into the background with the average similarity of about 90%.