FENG Ai-ming, FANG Li-min, LIN Min. Gaussian Process Regression and Its Application in Near-Infrared Spectroscopy Analysis[J]. Spectroscopy and Spectral Analysis, 2011, 31(6): 1514

Search by keywords or author
- Spectroscopy and Spectral Analysis
- Vol. 31, Issue 6, 1514 (2011)
Abstract
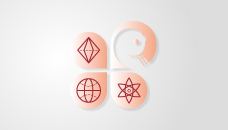
Set citation alerts for the article
Please enter your email address