ZHANG Jian-nan, WU Fu-chao, LUO A-li. Kernel Regression Application in Estimating Stellar Fundamental Parameters[J]. Spectroscopy and Spectral Analysis, 2009, 29(4): 1131

Search by keywords or author
- Spectroscopy and Spectral Analysis
- Vol. 29, Issue 4, 1131 (2009)
Abstract
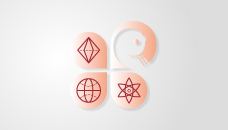
Set citation alerts for the article
Please enter your email address