
- Photonics Research
- Vol. 11, Issue 2, 212 (2023)
Abstract
1. INTRODUCTION
Digital cameras are ubiquitous in our daily life. For instance, they are the essential components of mobile phones and have been designed to accommodate a range of functions, such as photography, 3D time-of-fight (ToF) ranging and biometric recognition. As a sensing platform, more and more digital cameras tend to be integrated into the mobile phones to unlock more utilities. There are majorly two types of digital cameras, which are monochromatic/gray cameras and RGB cameras. In the latter, a Bayer filter array is integrated with the camera sensor chip to obtain three spectral channels. However, both types are incapable of imaging the scene with more spectral channels, i.e., multispectral imaging, which is crucial for a plethora of applications, such as combustion diagnostics [1–3], cancer detection [4], remote sensing [5,6], medical diagnostics [7,8], pollution detection [9], and agricultural applications [10,11]. Because of this, multispectral imaging has attracted enormous attention in the past decades. It essentially relates to the recovery of a data cube
To overcome the aforementioned limitation, the so-called snapshot spectral imaging techniques have been developed based on which the data cube can be computationally recovered from a 2D spatially and spectrally encoded image captured within a single exposure. For example, Gehm
The so-called computed tomography imaging spectrometry (CTIS) is another type of snapshot spectral imaging technique that shares the same principle as X-ray CT, which is a commercially mature technique and enjoys enormous amount of reconstruction algorithms that have been developed during the past half century [19–21]. However, CTIS suffers from a low spatial resolution as the imaging sensor is divided into an array of regions, each of which contains the projection of the target data cube along a distinct angle. Also, there are severe artifacts in the reconstruction due to the limited number of projections and the minor angular difference between them. This is also referred to as the missing-cone problem in the literature [22].
Sign up for Photonics Research TOC. Get the latest issue of Photonics Research delivered right to you!Sign up now
In order to improve its performance, extensive efforts have been devoted to either optimizing the optical components or the reconstruction algorithms. In terms of optical components, most researchers focused on the optimal design of the disperser as it determines the number of projections, the angular difference, and the energy distribution among them. Descour [23] distributed effectively the incident spectrum over the detector array by designing the original disperser composed of three cross cosine gratings, which had the problem of misalignment between the gratings and low diffraction efficiency of the higher-order projections. Volin
With respect to reconstruction algorithms, improving reconstruction accuracy and convergence speed is the major research interests. The most widely adopted reconstruction algorithms so far are the multiplicative algebraic reconstruction technique [27] and the expectation maximization (EM) [28], which have advantages including easy implementation and fast convergence [29]. Based on the noise source of the actual imaging system, Garcia and Dereniak [30] combined Poisson-distributed photon noise in the image and signal-independent system noise by using the standard maximum likelihood to design a mixed-expectation reconstruction technique that can effectively mitigate the influence of noise. An
In this paper, we develop a super-resolution CTIS (SRCTIS) by combining a conventional CTIS system and an RGB camera. The former can reconstruct a multispectral data cube with a low spatial resolution, and the latter can capture an RGB data cube with a high spatial resolution. In order to effectively assimilate the information of both data cubes, we first introduce GIF into each iteration of the CTIS reconstruction process to reduce severe artifacts due to the limited number of projections and angle span. The multispectral data cube is then mapped onto the RGB image through camera calibration. Finally, according to the spectral and the spatial continuity of the sought target, the multispectral information is propagated to each RGB pixel by applying the spectral propagation algorithm to obtain an image with a high resolution in both the spectral and the spatial domains. Different from aforementioned hybrid systems, which sample multispectral pixels at sparsely distributed locations, our hybrid system relies on CTIS which can recover the multispectral information of all pixels within a continuous region. Thus, it can be applied to scenes with more complex spatial structures. In addition, it can effectively avoid the problem of metamerism. The details of the proposed technique along with the simulative studies and proof-of-concept experiments are discussed in the following sections.
2. MATHEMATICAL FORMULATION AND RECONSTRUCTION
A. Modeling of the Forward Process
Figure 1 illustrates the layout of the proposed SRCTIS system, which includes two arms, i.e., a conventional CTIS system and an RGB camera. The incoming spectral image is first filtered with a filter to truncate the spectral information outside of the target range. The image is then divided into two identical parts, each delivered along one arm. The CTIS arm consists of an objective lens, a collimation lens, a diffractive optical element (DOE), and a gray camera. The objective lens collects the filtered spectral image, and the collimation lens then converts the image into planar waves. The DOE is used to diffract each incoming monochromatic planar wave toward a
Figure 1.Schematic of the SRCTIS system. The input image is split into two by a beam splitter and collected by an RGB camera and a conventional CTIS system, respectively. In the CTIS branch, after collimation, the input image is diffracted by the DOE and received by a gray camera.
Figure 2 illustrates the principle of SRCTIS for the generation of a data cube (i.e., a discretized version of the target spectral image) with both high spatial and spectral resolutions. The target data cube is diffracted toward the detector array along nine viewing angles, forming the same number of 2D projections. This forward imaging process can be mathematically described in a matrix form as
Figure 2.Principle of SRCTIS for reconstruction of a data cube with both high spatial and spectral resolutions. First, the CTIS reconstruction and an RGB image are obtained separately; then the CTIS multispectral pixels and the RGB image pixels are aligned by position calibration; and finally, a spectral propagation algorithm is used to fuse the two images.
B. Reconstruction Algorithm
Typically Eq. (1) is an ill-posed linear equation system, which can be solved with numerous mathematical techniques, such as optimization, iterative reconstruction, and machine learning algorithms. The gradient-based optimization methods are efficient but can be easily trapped in local minima; and the global optimizers, such as simulated annealing [37,38] and genetic algorithms [39], suffer from formidable computational costs. In addition, the machine learning algorithms usually require a large set of high-quality training data samples, which are not readily available [40–42]. Due to the ease of implementation and good performance for limited-projection tomography, a well-established iterative algorithm, i.e., the maximum likelihood expectation maximization (MLEM) [43], is adopted, and its iteration process can be described as
As can be seen from Fig. 2, the CTIS branch can recover more spectral details but has a lower spatial resolution, whereas, the RGB branch can capture more spatial information but with only three broadband spectral channels. The assimilation of the two data cubes can lead to the enhancement of the resolution in both the spectral and the spatial dimensions. For this purpose, we propose a hybrid algorithm that combines tomographic reconstruction aided with GIF [44] and a spectral propagation algorithm [45]. The first step of the method is a regular CTIS reconstruction but with an additional GIF step added within each MLEM iteration, and the corresponding flow chart is shown in Fig. 3. The reconstructed multispectral data cube is then mapped to the RGB data cube according to the geometrical relationship established based on camera calibration. Finally, the spectral resolution of the RGB image is enhanced by propagating the multispectral details mapped to the specific RGB pixels to their contagious RGB pixels through the spectral propagation algorithm, which is guided by the continuity in both the spatial and the spectral domains. The continuity condition plays a critical role for the successful implementation of the method. However, the conventional CTIS reconstruction usually suffers from severe artifacts mainly due to the insufficient number of projections and their minor angular difference, which would jeopardize the continuity condition and undermine the subsequent propagation process. To mitigate the limitations and take the full advantage of the information provided by the zero-order diffraction of the CTIS system, a smoothing operation, i.e., GIF is introduced into each iteration step to better preserve the edge information in the spatial domain. The filtered image
Figure 3.Flowchart of the MLEM algorithm with GIF. After each MLEM iteration, GIF is applied to suppress the reconstruction artifacts.
The second step of the hybrid algorithm is to align the multispectral image recovered by the CTIS branch to the RGB image. Considering the different magnifications of the two branches, a pixel in the multispectral image essentially corresponds to a certain area in the RGB image. To make an accurate matching, we first approximately determine the center positions of the multispectral pixels on the RGB image according to the camera calibration. Then, the squares, each centered at those positions with a side length of magnification, are considered to be the corresponding area for each multispectral pixel in the RGB image as indicated by a green square in Fig. 4(b). Furthermore, we take the spectral angle mapping (SAM) [46] as the quantitative indicator for positioning of the multispectral pixels, which is commonly applied to measure the similarity between two spectra. SAM treats the spectra as vectors whose dimensions equal the number of spectral bands and can be mathematically described as
Figure 4.(a) Calibrated RGB response curves; (b) projection of the CTIS multispectral pixels on the RGB image. The yellow pixels have unknown multispectral but known RGB details, and the blue pixels have both known RGB and multispectral details.
In order to determine the exact position of the multispectral pixel on the RGB image, we first convert the multispectral spectrum into an RGB vector by integrating the spectrum over the RGB camera response curves [see Fig. 4(a)]. Then, the resulting RGB vector is used to calculate the SAM values for all the RGB pixels within the green square. The position where the SAM value is minimum is taken as the position of the multispectral pixel in the RGB image. Figure 4(b) illustrates the distribution of the multispectral pixels on the RGB image. The distribution of blue pixels represents the actual positions of the CTIS multispectral pixels on the RGB image after matching the pixel points. The yellow pixels represent the remaining RGB pixels for which the multispectral information can be inferred from the neighboring blue pixels based on the spectral and spatial continuity conditions using a spectral propagation algorithm [45].
The spectral propagation algorithm takes into account not only the distance between the pixels in the spatial domain, but also the distance in the RGB domain. Since each RGB pixel has three values, the spectral propagation is conducted for each of the RGB channels. As illustrated in Fig. 4(b), assuming the spatial dimension of the RGB image is
Based on the above spectral propagation principle, it is obvious that the spectral information transferred from each multispectral pixel to the target RGB pixel is determined by the spatial distance between them, the similarity of RGB vectors, and the intensity difference between the two pixels in the corresponding channel. The spatial distance and the RGB vector difference determine the weight factors
3. RESULTS AND DISCUSSION
To demonstrate the reliability and superiority of our proposed SRCTIS system, we compare it to the conventional CTIS system with both numerical simulations and proof-of-concept experiments. In this paper, MLEM is adopted to invert the 2D CTIS diffraction patterns to recover a multispectral data cube, which has a low spatial resolution. To illustrate the effectiveness of GIF in suppressing the reconstruction artifacts of CTIS as well as the capability of SRCTIS in obtaining multispectral images with a high spatial resolution, we took the reconstructions from MLEM, MLEM with GIF (denoted as MLEM_GIF), and SRCTIS for comparison. In addition to qualitative analysis, we performed a detailed comparison between the algorithms in terms of quantitative image quality metrics, such as SAM, average normalized root-mean-square error (RMSE) [47], average peak signal-to-noise ratio (PSNR), and average structural similarity index measurement (SSIM) [47]. SAM quantifies the similarity between two spectra, and a smaller SAM value indicates a better spectral fidelity. RMSE is adopted to quantify the difference between the ground truth and the reconstructed data cube. A smaller RMSE suggests a closer reconstruction to the ground truth. On the other hand, PSNR and SSIM are mainly used to quantify the consistency between the spatial details of the reconstruction and that of the ground truth. PSNR is a quantitative indicator commonly used to evaluate quality of image reconstruction, and SSIM reflects the similarity between the ground truth and the reconstruction by fully considering the image composition, such as brightness, contrast, and structure.
For numerical validation, we randomly selected 30 representative scenes with distinct spatial and spectral features from three data sets, i.e., cave dataset [48], hyperspectral images for local illumination in natural scenes 2015 dataset [49], and ICVL hyperspectral dataset [50]. The scenes in these datasets all have spectral resolutions of 10 nm but have different spectral ranges of 400–700 nm, 400–720 nm, and 400–1000 nm, respectively. The spectral range of the selected scenes is tailored to be 420–700 nm, and a wavelength step of 5 nm is achieved by linear interpolation in the spectral dimension. To mimic the practical situations, we artificially added Gaussian noise to the simulated CTIS projections to obtain an SNR of 25–50 dB. The dimension of the reconstructed data cube is assumed to be
Figure 5.(a) Ground truth and the corresponding reconstructions of different scenes (denoted in sequence from left to right as “flowers,” “oil painting,” “cloth,” and “toys”) from MLEM and MLEM_GIF with the quality metrics PSNR/SSIM/SAM labeled at the bottom of each reconstructed image; (b) the relationships between the noise level and the different reconstruction quality metrics.
For the need of SRCTIS simulation, we further constructed 2D diffraction patterns and the high-spatial-resolution RGB image for the CTIS and the RGB branch, respectively. On one hand, we down-sampled the multispectral data cubes of size
Figure 6 shows the spatial details of both the reconstructions from different algorithms and the corresponding ground truths. In order to better compare the spatial details among MLEM, MLEM_GIF, and SRCTIS, all reconstructions are integrated over each of the RGB channels and then presented in the RGB form, and the local details are enlarged and placed in the upper left corner of each image. In the CTIS branch, as the input image is diffracted toward nine different angles after passing the DOE; the spatial resolution is essentially sacrificed for an improved spectral resolution. Therefore, it can be seen from Fig. 6 that MLEM reconstruction obviously loses considerable spatial details compared with the ground truth, and the relatively lower PSNR and SSIM values further suggest its reduced reconstruction quality in the spatial dimension from a quantitative point of view. For MLEM_GIF, although it can effectively suppress the artifacts in the CTIS reconstructed image and improve the reconstruction quality, it basically has the same discretization with MLEM and cannot increase the spatial resolution of the reconstructed image. On the contrary, as can be clearly seen from the local magnification of images recovered by SRCTIS, they have almost identical spatial details to the ground truth, which is far superior to MLEM and MLEM_GIF. It is worth noting that when calculating the quantitative image quality metrics, SRCTIS takes the original image with a spatial size of
Figure 6.Comparison of the spatial details of different scenes (denoted in sequence from top to bottom as “lilies,” “ruined house,” and “roof”) reconstructed from MLEM, MLEM_GIF, and SRCTIS. The enlarged images in the upper left corner, respectively, represent randomly selected local areas in the corresponding scene.
In addition to the spatial details, we also randomly selected three points (marked A, B, and C) from different scenes as shown in Fig. 6 to assess the spectral reconstruction quality, and the relevant results are presented in Fig. 7(a). As can be seen, the spectra reconstructed by MLEM deviate significantly from the ground truth (indicated by a large SAM value) and are accompanied by considerable noise. However, the reconstructed spectra of MLEM_GIF closely approximate the ground truth, and the SAM value is also far less than that of MLEM, further suggesting that GIF cannot only suppress the artifacts, but also improves the spectral quality. For SRCTIS, although the SAM value is slightly reduced compared with MLEM_GIF after propagation, the recovered spectrum of SRCTIS is basically consistent with that of MLEM_GIF and in good agreement with the ground truth. The quality of the spectrum is much higher than that of MLEM. Figure 7(b) presents the quantitative image quality metrics and the computational time corresponding to the reconstruction results of 10 different scenes with a noise level of 25 dB. It can be seen that in all cases, the quantitative metrics of the multispectral images obtained by SRCTIS are similar to those of MLEM_GIF and are better than that of MLEM, fully demonstrating the superiority of SRCTIS. In terms of computational time, it can be seen that SRCTIS only needs slightly longer computational time than MLEM_GIF.
Figure 7.(a) Spectra for randomly selected points (marked A, B, and C) in Fig.
In order to further validate the effectiveness of SRCTIS, we built a hybrid system according to the layout shown in Fig. 1 for a proof-of-concept experimental demonstration. A long-wave pass filter with a cutoff wavelength of 425 nm and a short-wave pass filter with a cutoff wavelength of 650 nm are combined to tailor the spectral information of the target scenes. The DOE is a binary phase element, etched into a layer (thickness of
We quantitatively analyzed the spatial and spectral resolutions of SRCTIS, and the corresponding results are shown in Fig. 8. In order to assess the spatial resolution of these methods, a USAF 1951 resolution test chart was adopted in our experiment. The spatial resolution of CTIS, SRCTIS, and the simple up-scale of CTIS using the nearest-neighbor interpolation (NNI) [54], and the RGB camera were compared against each other using the modulation transfer function (MTF) as shown in Fig. 8(a). As can be seen from the figure, the MTF of NNI largely overlaps with that of CTIS, suggesting that the direct application of a simple up-scale method [54] cannot improve the spatial resolution. Also, the MTF of SRCTIS is very close to that of the RGB camera, indicating that SRCTIS can effectively inherit the spatial resolution of the latter. These results imply that SRCTIS has a significant advantage in spatial resolution over conventional CTIS. According to the Rayleigh criterion and taking 20% contrast as the threshold, the spatial resolutions of CTIS, SRCTIS, NNI, and the RGB camera are determined to be 1.63 lp/mm, 6.46 lp/mm, 1.63 lp/mm, and 6.77 lp/mm, respectively. In addition, to quantify the spectral resolution, these methods were tested by reconstructing a uniform square region whose spectrum contains two adjacent peaks that are separated with different spacing. Figures 8(b) and 8(c) present the reconstructed spectra with minimum spacing between the peaks that CTIS and SRCTIS can resolve, respectively. The peaks are assumed to be resolved if the valley between the peaks is smaller than 50% of the peak values. The results show that the spectral resolutions of SRCTI and CTIS are similar, i.e., 12 nm and 10 nm, respectively. Thus, SRCTIS can achieve a much better spatial resolution and a slightly lower spectral resolution than CTIS.
Figure 8.Quantitative comparison of different algorithms in terms of spatial and spectral resolutions. Panel (a) presents the modulation transfer functions corresponding to CTIS, SRCTIS, CTIS with the nearest-neighbor interpolation, and the RGB camera; panel (b) presents the reconstructed spectrum of CTIS where the reference spectrum contains two peaks at 495 nm and 505 nm, respectively; panel (c) presents the reconstructed spectrum of SRCTIS where the reference spectrum contains two peaks at 493 nm and 505 nm, respectively.
Figure 9 compares the reconstruction results from different algorithms and the ground truths. Figure 9(a) shows the spatial details of different scenes (denoted in sequence as “hills, sailboat, landscape painting, and blueberries) reconstructed from different algorithms. The high-resolution RGB images captured by the RGB branch are shown in the RGB form, and the reconstruction results of MLEM, MLEM_GIF, and SRCTIS are compared at the 610-nm wavelength band to fully illustrate the superiority of SRCTIS in the spatial domain. The exposure times for the four scenes are 13 ms, 9 ms, 13 ms, and 12 ms, respectively, and the corresponding SNRs are 26.85 dB, 29.99 dB, 29.62 dB, and 30.23 dB, respectively. It can be seen that the artifacts in the reconstructed image of MLEM_GIF are greatly suppressed compared to MLEM by applying GIF in the iterative reconstruction process, which once again verifies the advantage of GIF. It is worth noting that there are also the mosaiclike artifacts in the MLEM reconstruction, which are caused by dividing the target scene into
Figure 9.(a) Experimental reconstructions of different scenes (denoted in sequence from top to bottom as “hills,” “sailboat,” “landscape painting,” and “blueberries”) from MLEM, MLEM_GIF, and SRCTIS, respectively; (b) reconstruction results of the hills scene at different wavelengths under SRCTIS; (c) spectra of randomly selected points (marked A, B, C, and D) from different scenes shown in panel (a).
In addition, the reconstructions from SRCTIS also have a higher spectral fidelity compared with those from MLEM. Four random points (marked A, B, C, and D) in different scenes as shown in Fig. 9(a) are selected and their corresponding spectra are shown in Fig. 9(c). It can be seen that, although the SRCTIS results are derived based on the MLEM_GIF reconstructions, the spectral information is not severely impaired by the propagation process and closely approximates the ground truth. Compared with MLEM, its spectra can better capture the variations and peak positions of the real spectrum. These results firmly suggest that SRCTIS can effectively inherit the spectral details of CTIS reconstructions optimized by GIF.
4. CONCLUSIONS AND OUTLOOK
In this paper, a super-resolution CTIS, which was capable of capturing images with both high spatial and spectral resolutions, has been developed and verified by both numerical simulations and proof-of-concept experiments. In the SRCTIS system, the complementary information was collected from both the CTIS and the RGB branches. For the CTIS reconstruction, we took full advantage of the zero-order pattern as the guidance image for filtering within each iterative step to effectively suppress the reconstruction artifacts and improve the reconstruction quality. After mapping the multispectral information reconstructed to the RGB image, which had a finer spatial resolution, we further propagated the multispectral information to each pixel in the RGB image based on the continuity condition in both the spectral and the spatial dimensions. The results from both the simulative and the proof-of-concept experiments suggested that SRCTIS can inherit the advantages of both the CTIS and the RGB branches and fulfill the high-quality reconstruction performance. Compared with the conventional CTIS system, the SRCTIS system could not only effectively suppress artifacts, but also greatly improve the spatial resolution, whereas, maintaining a high spectral fidelity.
Finally, we would like to point out some future research directions to further improve our method. The optimization of DOE effectively improved the intensity uniformity between different diffraction orders at the entire working wavelength range and improved the transmission efficiency of DOE, so as to obtain a more stable and well-behaved weight matrix and reduce the impact of noise to make reconstruction more accurate. In addition, the application of super-resolution and denoising neural networks in CTIS reconstruction effectively improved the efficiency as well as simplified the structure of the entire optical system.
References
[7] G. Lu, B. Fei. Medical hyperspectral imaging: a review. J. Biomed. Opt., 19, 010901(2014).
[21] P. C. Hansen. Rank-Deficient and Discrete Ill-Posed Problems: Numerical Aspects of Linear Inversion(1998).
[22] J. F. Scholl. The design and analysis of computed tomographic imaging spectrometers (CTIS) using Fourier and wavelet crosstalk matrices(2010).
[23] M. R. Descour. Non-scanning imaging spectrometry(1994).
[27] C. E. Volin. Portable snapshot infrared imaging spectrometer(2000).
[28] T. K. Moon. The expectation-maximization algorithm. IEEE Signal Process Mag., 13, 47-60(1996).
[34] Y. Fu, Y. Zheng, I. Sato, Y. Sato. Exploiting spectral-spatial correlation for coded hyperspectral image restoration. IEEE Conference on Computer Vision and Pattern Recognition (CVPR), 3727-3736(2016).
[43] K. M. Busa, J. C. McDaniel, M. S. Brown, G. S. Diskin. Implementation of maximum-likelihood expectation-maximization algorithm for tomographic reconstruction of TDLAT measurements. 52nd Aerospace Sciences Meeting, 2014-0985(2014).
[50] B. Arad, O. Ben-Shahar. Sparse recovery of hyperspectral signal from natural RGB images. Computer Vision–ECCV, 19-34(2016).
[51] D. Bodenham. Adaptive estimation with change detection for streaming data(2014).
[54] V. Siddharth, S. H. Saeed, H. Dua. Image standardisation using interpolation. Int. J. Enhanc. Res. Sci. Technol. Eng., 4, 272-278(2015).
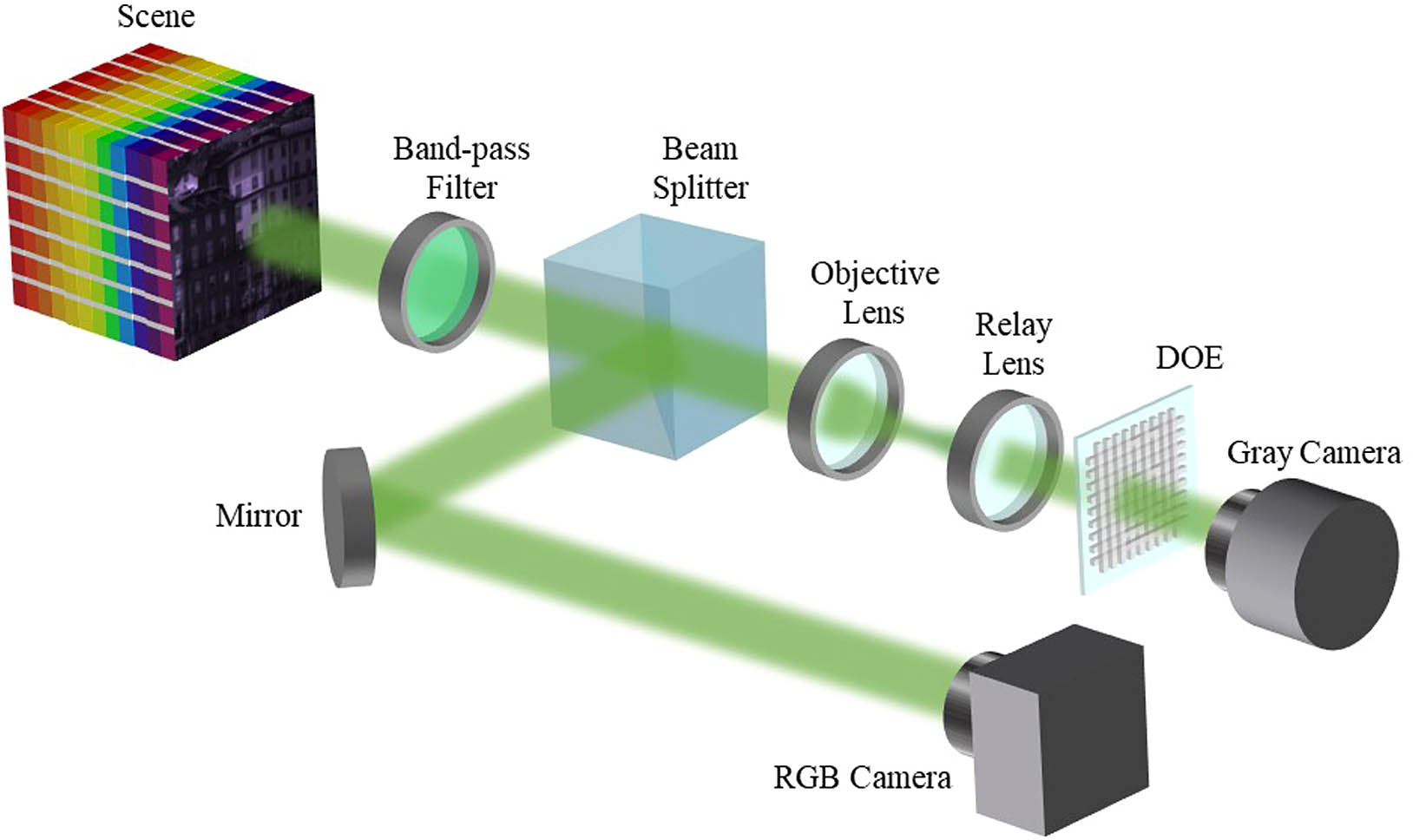
Set citation alerts for the article
Please enter your email address