LI Kai-yu, ZHANG Hui, MA Jun-cheng, ZHANG Ling-xian. Segmentation Method for Crop Leaf Spot Based on Semantic Segmentation and Visible Spectral Images[J]. Spectroscopy and Spectral Analysis, 2023, 43(4): 1248

Search by keywords or author
- Spectroscopy and Spectral Analysis
- Vol. 43, Issue 4, 1248 (2023)
Abstract
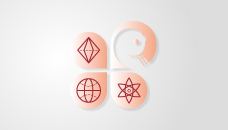
Set citation alerts for the article
Please enter your email address