LI Hang-fei, TU Liang-ping, HU Yu-han, LIU Hao, ZHAO Jian. Automatic Measurement of Stellar Atmospheric Physical Parameters Based on Kernel Ridge Regression Method[J]. Spectroscopy and Spectral Analysis, 2020, 40(4): 1297

Search by keywords or author
- Spectroscopy and Spectral Analysis
- Vol. 40, Issue 4, 1297 (2020)
Abstract
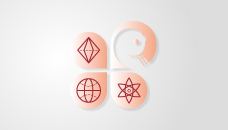
Set citation alerts for the article
Please enter your email address