Wen XIAO, Ying-li CAO, Shuai FENG, Ya-di LIU, Kai-lun JIANG, Zheng-xin YU, Li YAN. Detection of Rice Sheath Blight Disease Index Based on Split-Window Gram-Schmidt Transformation and PSO-SVR Algorithm[J]. Spectroscopy and Spectral Analysis, 2021, 41(7): 2181

Search by keywords or author
- Spectroscopy and Spectral Analysis
- Vol. 41, Issue 7, 2181 (2021)
Abstract
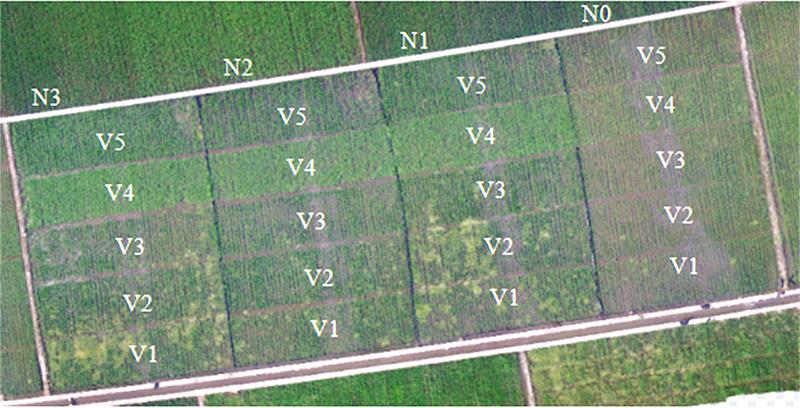
Set citation alerts for the article
Please enter your email address