MA Qian, YANG Wan-qi, LI Fu-sheng, CHENG Hui-zhu, ZHAO Yan-chun. Research on Classification of Heavy Metal Pb in Honeysuckle Based on XRF and Transfer Learning[J]. Spectroscopy and Spectral Analysis, 2023, 43(9): 2729

Search by keywords or author
- Spectroscopy and Spectral Analysis
- Vol. 43, Issue 9, 2729 (2023)
Abstract
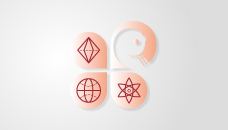
Set citation alerts for the article
Please enter your email address