WU Ting-ting, YU Ke-qiang, ZHANG Hai-hui, FENG Yi, ZHANG Xiao, WANG Hui-hui. Optimized Detection Models for Wheat Black Tip Disease and Multiple Classification Results[J]. Spectroscopy and Spectral Analysis, 2018, 38(12): 3912

Search by keywords or author
- Spectroscopy and Spectral Analysis
- Vol. 38, Issue 12, 3912 (2018)
Abstract
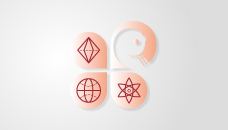
Set citation alerts for the article
Please enter your email address